End-to-end speech recognition method and system and storage medium
A speech recognition and speech feature technology, applied in speech recognition, speech analysis, neural learning methods, etc., can solve the problems of incomparable recognition effect, deviation of model to data fitting, poor noise robustness, etc.
- Summary
- Abstract
- Description
- Claims
- Application Information
AI Technical Summary
Problems solved by technology
Method used
Image
Examples
Embodiment 1
[0033] refer to figure 2 , this embodiment provides an end-to-end speech recognition method, the method includes the following steps:
[0034] S1: Train the initial speech feature extraction model;
[0035] Specifically, in this embodiment, pre-training is performed on a source corpus (a corpus with sufficient data, usually referring to the amount of training data > 100 hours), and the initial weight value of the model is obtained. The source corpus can choose a public corpus, such as THCHS-30, Aishell, ST-CMDS;
[0036] The deep CNN can enhance the representation of the frequency domain features of the speech signal. Therefore, the VGGNet model architecture can be used for the extraction of speech features. In this embodiment, the VGG16 model is used to extract the frequency domain features of the speech signal. Refer to image 3 , is a schematic diagram of the structure of the VGG16 model. The VGG16 model consists of 13 convolutional layers and 3 fully connected layers. A...
Embodiment 2
[0062] This embodiment provides an end-to-end speech recognition system, refer to Figure 5 , the system includes the following modules:
[0063] The model training module 101 is used to train an initial speech feature extraction model using the source corpus based on the VGGNet model, remove the fully connected layer in the initial speech feature extraction model and freeze a preset number of convolutional layer parameters, and use the target corpus The initial speech feature extraction model after removal and freezing is trained to obtain a frequency domain feature extraction network; it should be noted here that, due to the specific model training process, steps S1-S2 of the end-to-end speech recognition method in Embodiment 1 are performed. It has been elaborated in detail, so it will not be repeated here.
[0064] The framework building module 102 is used for constructing an end-to-end speech recognition framework. After the frequency domain feature extraction network, a...
PUM
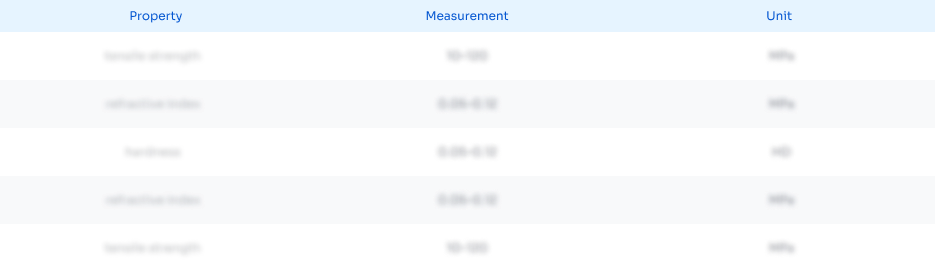
Abstract
Description
Claims
Application Information
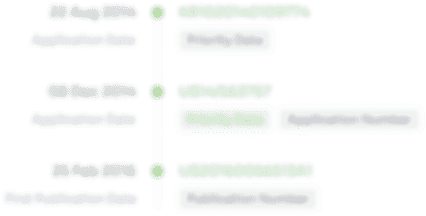
- R&D Engineer
- R&D Manager
- IP Professional
- Industry Leading Data Capabilities
- Powerful AI technology
- Patent DNA Extraction
Browse by: Latest US Patents, China's latest patents, Technical Efficacy Thesaurus, Application Domain, Technology Topic, Popular Technical Reports.
© 2024 PatSnap. All rights reserved.Legal|Privacy policy|Modern Slavery Act Transparency Statement|Sitemap|About US| Contact US: help@patsnap.com