Video semantic segmentation network training method, system and device and storage medium
A semantic segmentation and training method technology, applied in the field of video analysis, can solve the problems of not fully mining the characteristics of video data, the impact of model generalization performance, and the inability to effectively use video data, so as to improve generalization performance and alleviate frame-to-frame oversimulation Combined phenomenon, the effect of high segmentation accuracy
- Summary
- Abstract
- Description
- Claims
- Application Information
AI Technical Summary
Problems solved by technology
Method used
Image
Examples
Embodiment 1
[0044] An embodiment of the present invention provides a training method for a video semantic segmentation network, figure 2 The main flow of the method is shown, image 3 The general framework of the method is shown; the method mainly includes:
[0045] 1. Obtain training video data including several video clips.
[0046] There are two types of video clips, one is a video clip containing labeled frame images and unlabeled frame images, and the other is a video clip containing only unlabeled frame images, called unlabeled video clips.
[0047] Among them, the setting method of the marked frame in the video segment containing marked frame images and unmarked frame images can be realized by referring to conventional technology. Taking the typical public dataset Cityscapes as an example, the 20th frame is marked for every 30 frames. image 3 Unlabeled frame image in frame image with annotation subscript t 2 with t 1 Represents different moments, which can be adjacent mom...
Embodiment 2
[0106] The present invention also provides a training system for a video semantic segmentation network, which is mainly implemented based on the method provided in the first embodiment, as Figure 4 As shown, the system mainly includes:
[0107] The data acquisition unit is used to obtain training video data that includes several video clips; the video clips include labeled frame images and unlabeled frame images, or only include unlabeled frame images; when only unlabeled frame images are included in the video clips, from A single image is sampled from the video clip and a pseudo-label is obtained through feature extraction and classification, and the corresponding image is used as an annotated frame image;
[0108] The category prototype generation unit is used to input the unlabeled frame image to the video semantic segmentation network to be trained for feature extraction and classification, the classification result is used as a pseudo-label, and the category prototype of...
Embodiment 3
[0116] The present invention also provides a processing device, such as Figure 5 As shown, it mainly includes: one or more processors; memory for storing one or more programs; wherein, when the one or more programs are executed by the one or more processors, the One or more processors implement the methods provided in the foregoing embodiments.
[0117] Further, the processing device further includes at least one input device and at least one output device; in the processing device, the processor, memory, input device, and output device are connected through a bus.
[0118] In the embodiment of the present invention, the specific types of the memory, input device and output device are not limited; for example:
[0119] The input device can be a touch screen, an image acquisition device, a physical button or a mouse, etc.;
[0120] The output device can be a display terminal;
[0121] The memory may be random access memory (Random Access Memory, RAM), or non-volatile memory...
PUM
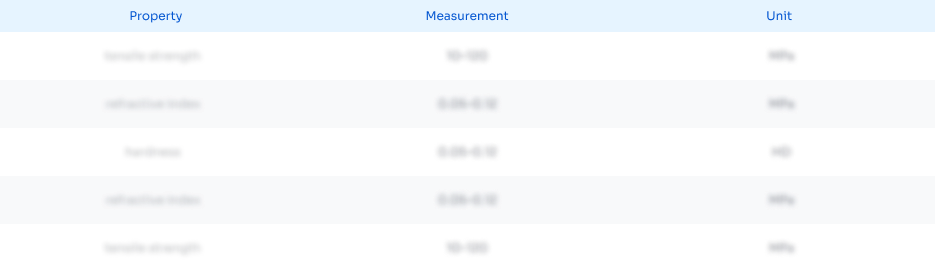
Abstract
Description
Claims
Application Information
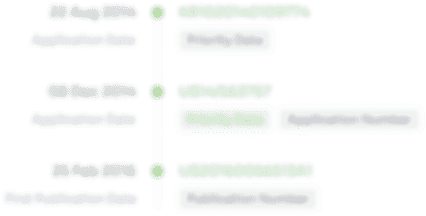
- R&D Engineer
- R&D Manager
- IP Professional
- Industry Leading Data Capabilities
- Powerful AI technology
- Patent DNA Extraction
Browse by: Latest US Patents, China's latest patents, Technical Efficacy Thesaurus, Application Domain, Technology Topic, Popular Technical Reports.
© 2024 PatSnap. All rights reserved.Legal|Privacy policy|Modern Slavery Act Transparency Statement|Sitemap|About US| Contact US: help@patsnap.com