Method for reducing dimensions of hyper-spectral data on basis of pairwise constraint discriminate analysis and non-negative sparse divergence
A discriminant analysis and non-negative sparse technology, which is applied in the field of hyperspectral remote sensing image processing, can solve the problems of reduced migration efficiency, kernel matrix without discriminant information, and high computational overhead.
- Summary
- Abstract
- Description
- Claims
- Application Information
AI Technical Summary
Problems solved by technology
Method used
Image
Examples
Embodiment 1
[0092] Example 1: A hyperspectral data dimensionality reduction method based on pairwise constraints discriminative analysis-non-negative sparse divergence (pairwise constraints discriminative analysis-non-negative sparse divergence, PCDA-NSD), the dimensionality reduction method is aimed at following the target With the increase of hyperspectral data, there are fewer and fewer source hyperspectral data that can be directly used, and when the source hyperspectral data and target hyperspectral data come from different distributions, the classification performance of many advanced machine learning-based hyperspectral data classification algorithms worse. First, based on a pair-constrained sample that can automatically obtain discriminant information, a pair-constrained discriminant analysis is proposed; then, a non-negative sparse divergence criterion is designed to construct the relationship between source and target hyperspectral data with different distributions. Finally, com...
Embodiment 2
[0152] Embodiment 2: Through real hyperspectral data (Hyperion Botswana, AVIRIS KSC, AVIRIS 92AV3C and ProSpecTIR ACER) experiment, PCDA-NSD of the present invention is classified with existing TSSL-MMD, TCA, STME, PCA dimensionality reduction algorithm and SVM Algorithms were compared. For the fairness of the comparison, SVM (Support Vector Machine, Support Vector Machine) was uniformly used for supervised classification. The kernel function of SVM was a Gaussian kernel function, and the width and penalty factor of the kernel function were obtained by 5-fold cross-validation. In order to eliminate the influence of random factors, each experiment was done 20 times and the average value was taken. Prove the superiority of PCDA-NSD.
[0153] combine figure 1 , the figure shows the key steps of using the PCDA-NSD method for dimensionality reduction and classification of hyperspectral data, which mainly includes four steps: first: select the source field and target field hyperspe...
PUM
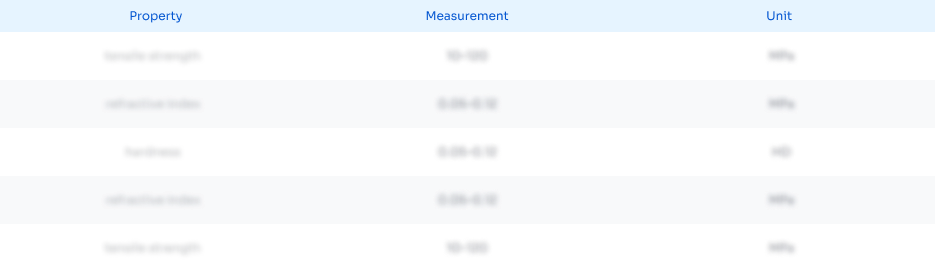
Abstract
Description
Claims
Application Information
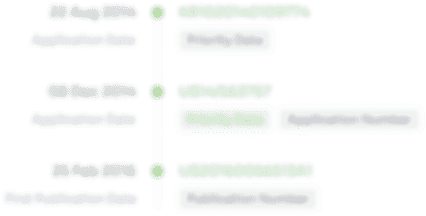
- Generate Ideas
- Intellectual Property
- Life Sciences
- Materials
- Tech Scout
- Unparalleled Data Quality
- Higher Quality Content
- 60% Fewer Hallucinations
Browse by: Latest US Patents, China's latest patents, Technical Efficacy Thesaurus, Application Domain, Technology Topic, Popular Technical Reports.
© 2025 PatSnap. All rights reserved.Legal|Privacy policy|Modern Slavery Act Transparency Statement|Sitemap|About US| Contact US: help@patsnap.com