Reality scene image segmentation method based on contrast self-supervised learning
A supervised learning and image segmentation technology, applied in neural learning methods, image analysis, image data processing, etc., can solve the problems of ignoring the relationship, not considering the loss of spatial continuity, and limiting the generalization ability of the model to image segmentation. Generalization ability, the effect of reducing labor cost
- Summary
- Abstract
- Description
- Claims
- Application Information
AI Technical Summary
Problems solved by technology
Method used
Image
Examples
Embodiment Construction
[0044] The technical solutions of the present invention will be described in detail below in conjunction with the drawings and specific embodiments, but this does not limit the protection scope of the present application.
[0045] The present invention is a kind of real scene image segmentation method based on comparative self-supervised learning, and the method comprises the following contents:
[0046] 1. Design comparison self-supervised segmentation module:
[0047] Contrastive self-supervised learning segmentation model includes upper and lower branches with the same structure, each branch includes sequentially connected encoder, decoder, feature projection module and predictor; the input image is randomly cropped into two overlapping regions Image patches, two image patches are respectively processed by the two branches of the contrastive self-supervised learning segmentation model;
[0048] The encoder includes a deep convolutional neural network and a spatial pyramid ...
PUM
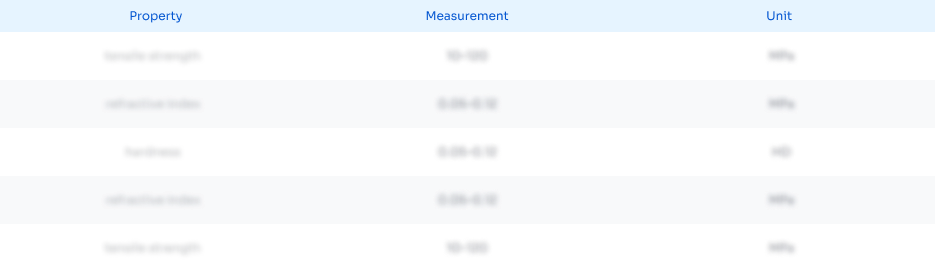
Abstract
Description
Claims
Application Information
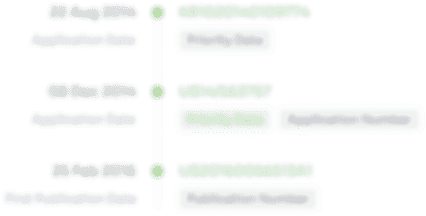
- Generate Ideas
- Intellectual Property
- Life Sciences
- Materials
- Tech Scout
- Unparalleled Data Quality
- Higher Quality Content
- 60% Fewer Hallucinations
Browse by: Latest US Patents, China's latest patents, Technical Efficacy Thesaurus, Application Domain, Technology Topic, Popular Technical Reports.
© 2025 PatSnap. All rights reserved.Legal|Privacy policy|Modern Slavery Act Transparency Statement|Sitemap|About US| Contact US: help@patsnap.com