Lightweight target detection method
A target detection and lightweight technology, applied in neural learning methods, instruments, biological neural network models, etc., can solve the problems of increasing detection time and low detection accuracy, improving efficiency, reducing network parameters and calculation amount, and reducing network parameters. The effect of predicting time
- Summary
- Abstract
- Description
- Claims
- Application Information
AI Technical Summary
Problems solved by technology
Method used
Image
Examples
Embodiment Construction
[0029] The specific implementation, features and effects of a light-weight target detection method according to the present invention will be described in detail below with reference to the accompanying drawings and preferred embodiments.
[0030] see figure 1 , a lightweight target detection method of the present invention, wherein: the method comprises the following steps:
[0031]Step 1: Use the Mosaic method to perform data enhancement on the training image samples: mosaic data enhancement uses four images, and stitches the four images. Each image has its corresponding target frame. After stitching the four images, you can get A brand new image, and all the target frames corresponding to this image are also obtained, and the obtained new image is sent to the network for learning, which expands the data set and increases the background complexity of the data set.
[0032] First read the four images in the data set, respectively flip the four images (flip the original image...
PUM
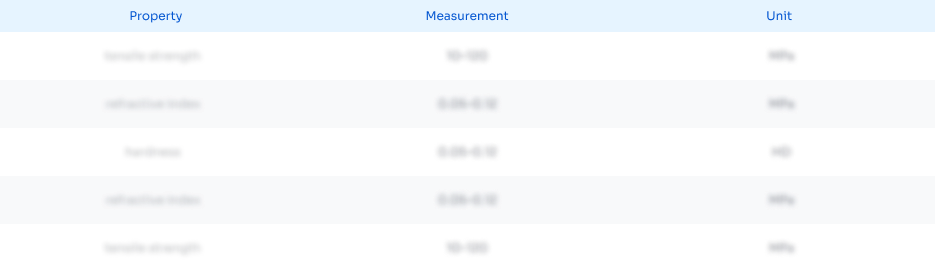
Abstract
Description
Claims
Application Information
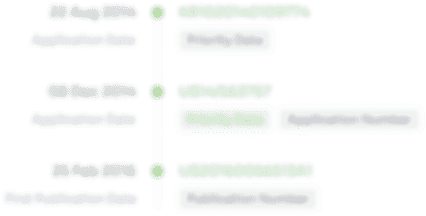
- R&D Engineer
- R&D Manager
- IP Professional
- Industry Leading Data Capabilities
- Powerful AI technology
- Patent DNA Extraction
Browse by: Latest US Patents, China's latest patents, Technical Efficacy Thesaurus, Application Domain, Technology Topic, Popular Technical Reports.
© 2024 PatSnap. All rights reserved.Legal|Privacy policy|Modern Slavery Act Transparency Statement|Sitemap|About US| Contact US: help@patsnap.com