Pavement defect detection method and system based on deep learning
A defect detection and deep learning technology, which is applied in neural learning methods, image data processing, image enhancement, etc., can solve the problems of single feature and reduce the amount of calculation, so as to reduce the amount of calculation, remove redundant information, and improve detection efficiency Effect
- Summary
- Abstract
- Description
- Claims
- Application Information
AI Technical Summary
Problems solved by technology
Method used
Image
Examples
Embodiment
[0041] The U-MDN designed for the present invention is composed of two parts of U-NET and U-MDM in the overall network structure. First use U-NET to extract multi-scale information from different convolutional blocks, and then use U-MDM to further extract deep features from four different sizes, and finally simultaneously fuse the shallow feature with deep features using U-NET. Finally, the prediction result is obtained through the Sigmoid function.
[0042] Division network design, U-NET depth neural networks are symmetrical U-shaped structures containing the lower sampling image compression path and the upper sampling image extension path. This structure is a basic idea of the FCN codec structure. And both networks use convolution operation instead of full connection operation, which greatly reduces the amount of network model parameters and improves the model training speed. Different, U-NET will focus on the upper sampling stage, increasing the number of Feature Map in the a...
PUM
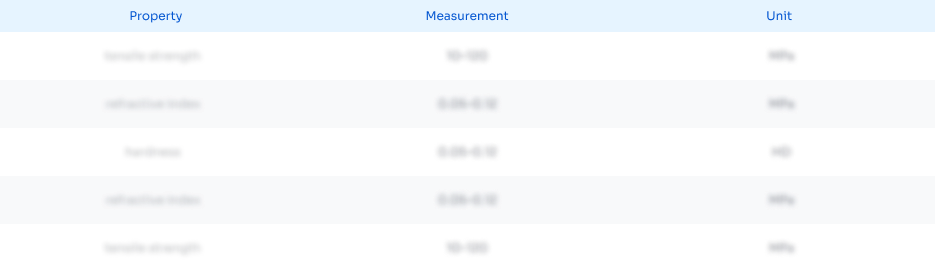
Abstract
Description
Claims
Application Information
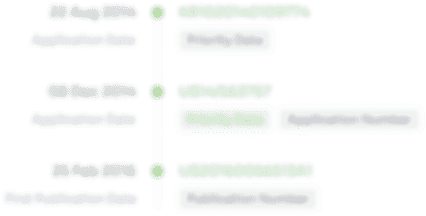
- Generate Ideas
- Intellectual Property
- Life Sciences
- Materials
- Tech Scout
- Unparalleled Data Quality
- Higher Quality Content
- 60% Fewer Hallucinations
Browse by: Latest US Patents, China's latest patents, Technical Efficacy Thesaurus, Application Domain, Technology Topic, Popular Technical Reports.
© 2025 PatSnap. All rights reserved.Legal|Privacy policy|Modern Slavery Act Transparency Statement|Sitemap|About US| Contact US: help@patsnap.com