Bearing fault diagnosis method based on cyclic correlation entropy and one-dimensional shallow convolutional neural network
A convolutional neural network and fault diagnosis technology, applied in the fields of deep learning and signal processing, can solve the problems of poor diagnosis effect and large amount of calculation of bearing signals processed by 1DCNN, so as to suppress non-Gaussian noise, simplify the structure, and avoid computational complexity. high effect
- Summary
- Abstract
- Description
- Claims
- Application Information
AI Technical Summary
Problems solved by technology
Method used
Image
Examples
Embodiment 1
[0042] Such as figure 1As shown, the present invention discloses a bearing fault diagnosis method of cyclic correlation entropy and one-dimensional shallow convolutional neural network, comprising the following steps:
[0043] Step 1, collect N vibration signal samples Each sample signal x i The number of sampling points is M, and the sampling frequency is f s ;
[0044] Step 2, calculate each signal sample x i The time-varying correlation entropy matrix of The calculation formula is: Where τ is the delay variable, E(·) is the expectation operator, σ is the kernel length of the kernel function, κ σ ( ) is a kernel function that satisfies the Mercer condition, and the kernel function κ σ (·) Using Laplace kernel function, its calculation formula is: Where exp(·) is an exponential function, and ||·|| is a norm operator.
[0045] Step 3, calculate each signal sample x i The circular correlation entropy spectral density matrix of The formula is: Among them, FFT2[...
Embodiment 2
[0050] The experimental verification is carried out on the rolling bearing data set of Case Western Reserve University (CWRU). The sampling frequency of the motor vibration signal is 48kHz. The faulty bearing is the driving end deep groove ball bearing. The bearing model is SKF6205. The faulty defect of the bearing is processed by EDM . The patent of the present invention divides the fault categories into 10 types. According to the defect position, it is divided into bearing rolling body damage, inner ring damage and outer ring damage. The three different damage diameters of 0.007inch, 0.014inch and 0.021inch correspond to slight, moderate and Severe damage, using data sets A, B, and C of 3 different speeds and loads of 1hp (1772 rpm), 2hp (1750 rpm) and 3hp (1730 rpm), bearing rolling elements, inner rings , outer ring damage and normal state are marked as BF7, BF14, BF21, IF7, IF14, IF21, OF7, OF14, OF21 and NC, respectively, as shown in Table 1.
[0051] generalized cyclos...
PUM
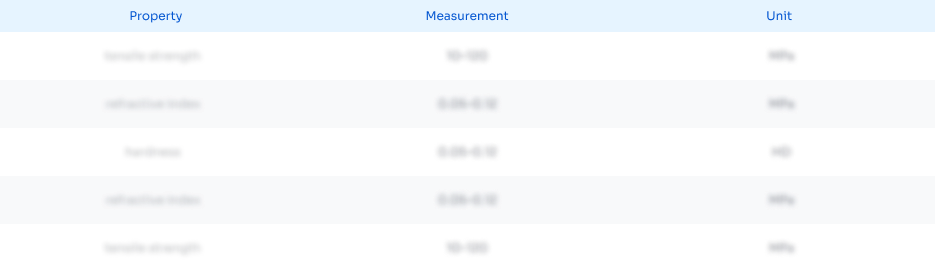
Abstract
Description
Claims
Application Information
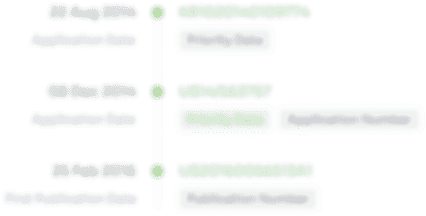
- R&D Engineer
- R&D Manager
- IP Professional
- Industry Leading Data Capabilities
- Powerful AI technology
- Patent DNA Extraction
Browse by: Latest US Patents, China's latest patents, Technical Efficacy Thesaurus, Application Domain, Technology Topic.
© 2024 PatSnap. All rights reserved.Legal|Privacy policy|Modern Slavery Act Transparency Statement|Sitemap