Target detection and identification method based on FSAF and fast-slow weight
A target detection and recognition method technology, applied in character and pattern recognition, instruments, biological neural network models, etc., can solve problems such as low average accuracy, improve accuracy and convergence speed, reduce computational complexity, and improve training accuracy Effect
- Summary
- Abstract
- Description
- Claims
- Application Information
AI Technical Summary
Problems solved by technology
Method used
Image
Examples
Embodiment 1
[0092] The present invention realizes the optimal selection of the feature layer based on the feature pyramid and RetinaNet network, combined with the fast-slow weight optimizer, there is a wide application space in underwater target recognition and target detection with fuzzy features in dark environments; in the marine industry, It has great practical significance in the application scenarios of fishery and land fuzzy environment. In the example, the simulated environment test is carried out on the training and testing data sets provided by the global underwater robot competition. Its data set is obtained from intercepting non-adjacent frames of videos in a simulated environment, and it has a good simulation of underwater blurred environments. In addition, it has achieved good results in classification and recognition data sets such as ImageNet, PASCAL VOC, and Labelme. Effect.
[0093] The configuration of the network model training and testing equipment used in the exampl...
Embodiment 2
[0186] This embodiment describes the specific implementation of the target detection and recognition method based on FSAF and fast-slow weights of the present invention, and realizes the performance comparison between the method and the RetinaNet network in the environment where the small data set is relatively clear, and shows two methods The comparison results of , including the following steps:
[0187] Step A. Divide the atlas used for comparing model effects into a training set with a number of 200 and an evaluation set with a number of 100, and the division method adopts random division;
[0188] During the specific implementation, the pictures come from the training and testing data sets provided by the underwater robot competition, and the data sets are obtained from the non-adjacent frames of the intercepted video in the simulated environment;
[0189] Step B, preprocessing the image;
[0190] Wherein graphics and processing are in order to obtain the image input mod...
PUM
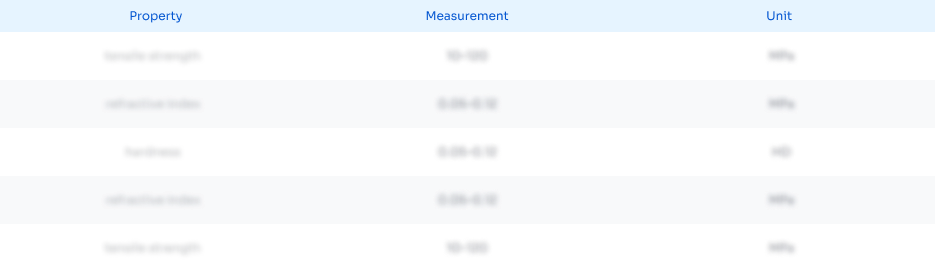
Abstract
Description
Claims
Application Information
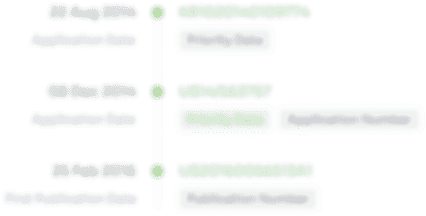
- R&D
- Intellectual Property
- Life Sciences
- Materials
- Tech Scout
- Unparalleled Data Quality
- Higher Quality Content
- 60% Fewer Hallucinations
Browse by: Latest US Patents, China's latest patents, Technical Efficacy Thesaurus, Application Domain, Technology Topic, Popular Technical Reports.
© 2025 PatSnap. All rights reserved.Legal|Privacy policy|Modern Slavery Act Transparency Statement|Sitemap|About US| Contact US: help@patsnap.com