Complex scene driving risk prediction method based on multiple time-space diagrams
A technology for risk prediction and complex scenarios, applied in neural learning methods, biological neural network models, neural architectures, etc., which can solve problems such as unfavorable applications, difficulties in building multi-vehicle collision risk prediction models, and failure to consider multi-vehicle collision risks. Achieve the effect of improving accuracy and practicality, and solving construction difficulties
- Summary
- Abstract
- Description
- Claims
- Application Information
AI Technical Summary
Problems solved by technology
Method used
Image
Examples
Embodiment Construction
[0084] The present invention will be further described below in conjunction with the accompanying drawings and specific embodiments, but the protection scope of the present invention is not limited thereto.
[0085] Such as figure 1 As shown, a complex scene driving risk prediction method based on multi-space-time graph, specifically includes the following steps:
[0086] Step 1, offline risk prediction model training
[0087] Take the self-vehicle and surrounding vehicles as the nodes in the graph at a certain moment, and take the vehicle position, velocity and acceleration as the node characteristics, and construct a node adjacency matrix reflecting different space-time relationships between vehicles, using the nodes in the graph, node characteristics and node adjacency matrix Obtain the multi-temporal-temporal graph describing the surrounding multi-vehicle complex scene, input the fused multi-temporal-temporal graph into the graph convolutional neural network, and extract ...
PUM
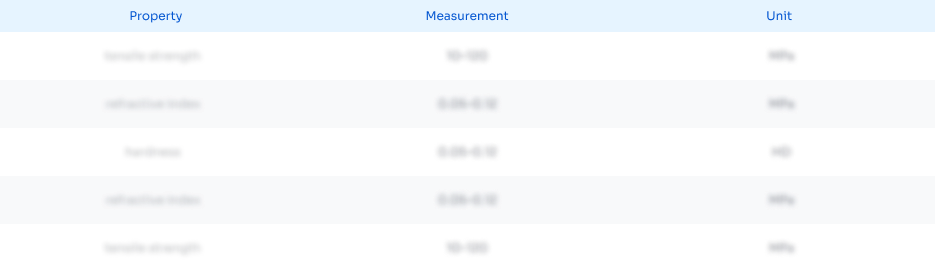
Abstract
Description
Claims
Application Information
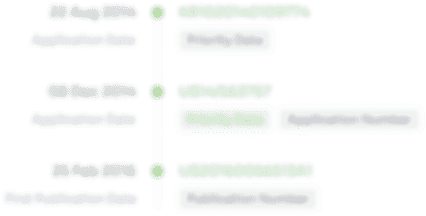
- Generate Ideas
- Intellectual Property
- Life Sciences
- Materials
- Tech Scout
- Unparalleled Data Quality
- Higher Quality Content
- 60% Fewer Hallucinations
Browse by: Latest US Patents, China's latest patents, Technical Efficacy Thesaurus, Application Domain, Technology Topic, Popular Technical Reports.
© 2025 PatSnap. All rights reserved.Legal|Privacy policy|Modern Slavery Act Transparency Statement|Sitemap|About US| Contact US: help@patsnap.com