RD time-frequency data-oriented deep learning model evaluation system and method
A deep learning and data technology, applied in the fields of artificial intelligence and aerospace, can solve problems such as no, and achieve the effect of reducing labor costs, accurate single-point optimization, and good generalization performance
- Summary
- Abstract
- Description
- Claims
- Application Information
AI Technical Summary
Problems solved by technology
Method used
Image
Examples
Embodiment Construction
[0135] Such as Figure 1-6 As shown, the present invention is a deep learning model evaluation system for RD time-frequency data, which is composed of a verification set expansion module, a multi-IOU threshold F1 score calculation module, a multi-IOU threshold mAP calculation module, a FLOPs calculation module, and an evaluation index integrated calculation module. .
[0136] The verification set expansion module receives the grayscaled training set and verification set, copy and paste blind detection tolerance, and expansion quantity as input, and analyzes the overall distribution of label positions in the overall data of the training set and verification set, including each The distribution of the number of targets in the image, the coordinate distribution of the upper left corner of the target, the distribution of the rotation angle of the target, and the distribution of the blind detection score of copy and paste, cut out the target in the image to form a target pool, and ...
PUM
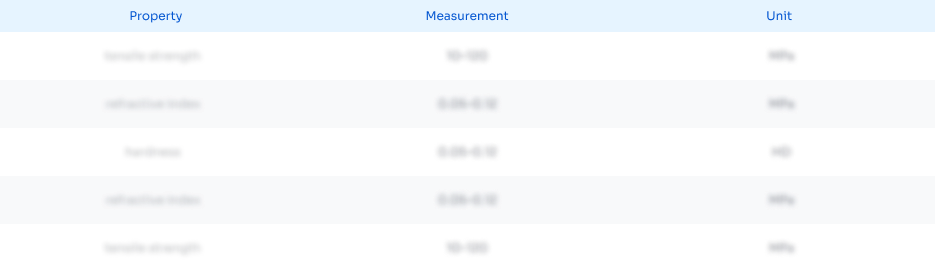
Abstract
Description
Claims
Application Information
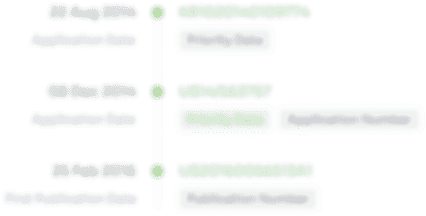
- Generate Ideas
- Intellectual Property
- Life Sciences
- Materials
- Tech Scout
- Unparalleled Data Quality
- Higher Quality Content
- 60% Fewer Hallucinations
Browse by: Latest US Patents, China's latest patents, Technical Efficacy Thesaurus, Application Domain, Technology Topic, Popular Technical Reports.
© 2025 PatSnap. All rights reserved.Legal|Privacy policy|Modern Slavery Act Transparency Statement|Sitemap|About US| Contact US: help@patsnap.com