Knowledge graph representation learning method based on entity and relation coding in neural network
A learning method and neural network technology, applied in biological neural network models, knowledge expression, neural architecture, etc., to achieve the effect of improving accuracy and learning accuracy
- Summary
- Abstract
- Description
- Claims
- Application Information
AI Technical Summary
Problems solved by technology
Method used
Image
Examples
Embodiment Construction
[0143] The present invention will be further described below.
[0144] A knowledge map representation learning method based on entity and relationship encoding in a neural network, comprising the following steps:
[0145] The first step is to construct the target triplet from the knowledge base, and obtain all the path relationships between the head entity and the tail entity in the triplet, the process is as follows:
[0146] 1.1. Obtain the entity set E and relation set R in the knowledge base, and construct a triplet S={(h,r,t)|h,t∈E∧r∈R}, r is the entity h and t The direct relationship between, h is the head entity, t is the tail entity;
[0147] 1.2. Obtain all relation path sets P={p between h and t 1 ,p 2 ,...p N}, where p i Represents the i-th path in the path set P, N represents the number of relationship paths, and the i-th path between h and t is denoted as P i =i1 ,r i2 ,...,r iM , t>, M represents the number of relationships on this relationship path;
[...
PUM
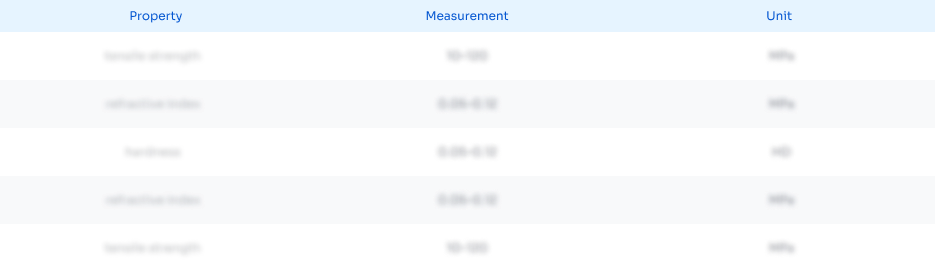
Abstract
Description
Claims
Application Information
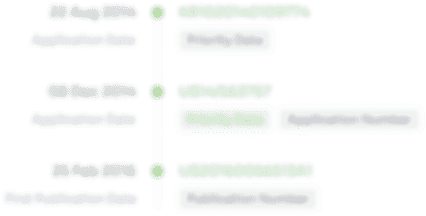
- R&D Engineer
- R&D Manager
- IP Professional
- Industry Leading Data Capabilities
- Powerful AI technology
- Patent DNA Extraction
Browse by: Latest US Patents, China's latest patents, Technical Efficacy Thesaurus, Application Domain, Technology Topic, Popular Technical Reports.
© 2024 PatSnap. All rights reserved.Legal|Privacy policy|Modern Slavery Act Transparency Statement|Sitemap|About US| Contact US: help@patsnap.com