Medical image target area identification method, neural network model and application
A technology of neural network model and target area, which is applied in the identification of medical image target area, neural network model and application fields, can solve the problems of many hyperparameters, slow reasoning speed, and long iteration cycle, so as to improve calculation speed and accuracy The effect of improving the degree of robustness
- Summary
- Abstract
- Description
- Claims
- Application Information
AI Technical Summary
Problems solved by technology
Method used
Image
Examples
Embodiment 1
[0052] This embodiment discloses a method for identifying a target area of an image. The image in this embodiment is introduced using an MRI image as an example, and the target area identified in this embodiment is introduced using a brain tumor in an MRI image as an example. The Dicom file includes MRI image weight information, and the target keyword is T2;
[0053] Include the following steps:
[0054] Step 1, traverse all Dicom files, use the open source tool PyDicom to read the sequence information of each Dicom file, and determine whether the sequence information contains the keyword T2, if the keyword T2 is included, then determine that the Dicom file is the target file, And use the PyDicom third-party library to read the matrix information in the Dicom file, and save the matrix information as an image in JPG format.
[0055] The format of the pictures taken by the nuclear magnetic resonance apparatus in the hospital is all Dicom format. The so-called Dicom format (D...
Embodiment 2
[0120] This embodiment discloses a neural network model based on a residual structure, including a shallow backbone network structure, a middle backbone network structure, a deep backbone network structure, an SPP layer, a first detection head, a second detection head, and a third detection head;
[0121] Each backbone network structure includes a residual structure, and the residual structure includes the basic unit formed by the first processing of the 1×1 Conv layer and the post-processing of the 3×3 Conv layer; in the same residual structure There is at least one basic unit; when the image to be recognized is processed by the shallow backbone network structure and input to the first detection head, it can output the target image with the first receptive field; when the image to be recognized is sequentially passed through the The shallow backbone network structure and the middle layer backbone network structure are processed and input to the second detection head, which can...
PUM
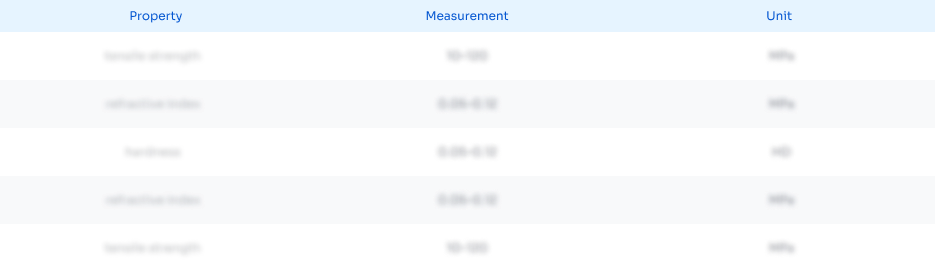
Abstract
Description
Claims
Application Information
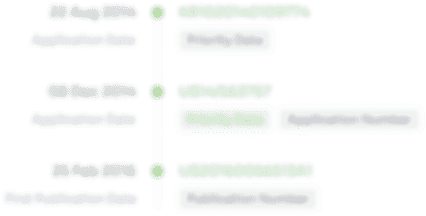
- R&D Engineer
- R&D Manager
- IP Professional
- Industry Leading Data Capabilities
- Powerful AI technology
- Patent DNA Extraction
Browse by: Latest US Patents, China's latest patents, Technical Efficacy Thesaurus, Application Domain, Technology Topic, Popular Technical Reports.
© 2024 PatSnap. All rights reserved.Legal|Privacy policy|Modern Slavery Act Transparency Statement|Sitemap|About US| Contact US: help@patsnap.com