Mixed model multivariate time sequence anomaly detection method based on graph neural network
A hybrid model and neural network technology, applied in biological neural network models, neural learning methods, neural architectures, etc., can solve the problem that abnormal time series detection cannot be simultaneously detected, abnormal time stamp detection, cannot be accurately detected, and cannot detect multiple time series. exception, etc.
- Summary
- Abstract
- Description
- Claims
- Application Information
AI Technical Summary
Problems solved by technology
Method used
Image
Examples
Embodiment 1
[0032] Specifically, in an embodiment of the present invention, based on the prediction model of the graph convolutional neural network, the graph G=(V, E) contains k nodes, each node represents a time series, has its own characteristics, and the edge represents The adjacency relationship between time series; the hidden representation of nodes is obtained by aggregating the adjacency features of nodes through the adjacency relationship of nodes, which is the process of graph convolution.
[0033] Specifically, in an embodiment of the present invention, a sliding window with a window size of w and a step size of 1 is used to generate H said first multivariate subsequences, where H=n-w+1; the i-th first A multivariate subsequence is denoted as X i =[x i ,...,x i+w-1 ]∈R k×w , where i={1,2,...,H}, x i Indicates the i-th column, taking the i-th column to the i+w-1th column of X as an example:
[0034] directly performing maximum and minimum normalization processing on each of...
PUM
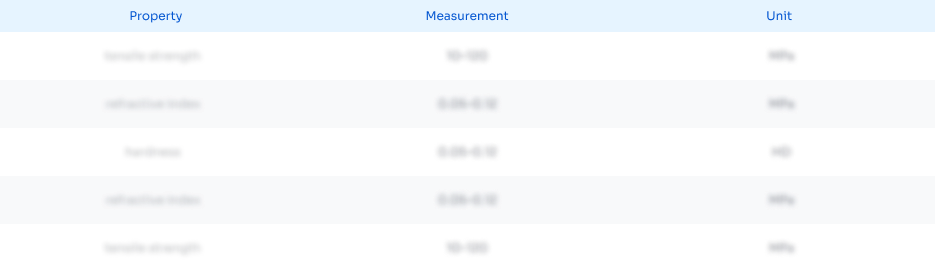
Abstract
Description
Claims
Application Information
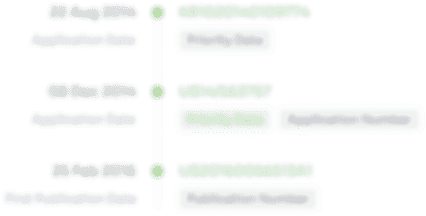
- R&D Engineer
- R&D Manager
- IP Professional
- Industry Leading Data Capabilities
- Powerful AI technology
- Patent DNA Extraction
Browse by: Latest US Patents, China's latest patents, Technical Efficacy Thesaurus, Application Domain, Technology Topic, Popular Technical Reports.
© 2024 PatSnap. All rights reserved.Legal|Privacy policy|Modern Slavery Act Transparency Statement|Sitemap|About US| Contact US: help@patsnap.com