Multi-modal fusion emotion recognition system and method based on multi-task learning and attention mechanism and experimental evaluation method
A multi-task learning and emotion recognition technology, applied in the field of human-computer interaction, can solve the problems of multi-modal emotion recognition process efficiency and low accuracy, and achieve the effect of improving calculation efficiency and accurate recognition
- Summary
- Abstract
- Description
- Claims
- Application Information
AI Technical Summary
Problems solved by technology
Method used
Image
Examples
specific Embodiment approach 1
[0045] Specific implementation mode 1: In this embodiment, by introducing multi-task learning and multi-modal emotion analysis, the multi-modal fusion emotion recognition process based on multi-task learning and attention mechanism is completed. The specific process is as follows:
[0046] First of all, multi-task learning (MTL) is a machine learning method that combines multiple tasks to learn at the same time. During the learning process, it helps each task to learn by using useful information between multiple related tasks. Obtain more accurate learning performance and enhance the representation and generalization capabilities of the model. The core of multi-task learning lies in the sharing of knowledge between tasks, so the challenge is the sharing mechanism between tasks. In deep learning, there are two sharing strategies, namely the hard sharing mechanism and the soft sharing mechanism of parameters, such as figure 2 As shown, the hard sharing mechanism shares hidden l...
specific Embodiment approach 2
[0096] Embodiment 2: According to the multi-modal fusion emotion recognition system and method based on multi-task learning and attention mechanism proposed in Embodiment 1, this embodiment proposes a multi-modal fusion emotion based on multi-task learning and attention mechanism Identify experimental analysis, process:
[0097] First, use the CMU-MOSI and CMU_MOSEI data sets for experimental simulation;
[0098] The content also comes from a certain website, including 22856 video clips and corresponding emotional tags. The range of emotional tags is [-3,+3]. for negative. The statistical information of the experimental data set is shown in Table 1:
[0099] Table 1 MOSI and MOSEI dataset information
[0100]
[0101] Secondly, regarding the experimental settings, it is written in python3.7.8 language and uses the deep learning framework pytorch1.4.0 to implement the neural network structure. The experimental environment is Windows10 system, and the experimental hardwar...
specific Embodiment approach 3
[0144] Specific Embodiment Three: According to the system and method provided in Embodiment 1 or 2, this embodiment divides the functional modules according to the block diagram shown in the accompanying drawings. For example, each functional module can be divided corresponding to each function, or two or More than two functions are integrated into one processing module; the above-mentioned integrated modules can be implemented in the form of hardware or in the form of software function modules. It should be noted that the division of modules in the embodiment of the present invention is schematic, and is only a logical function division, and there may be another division manner in actual implementation.
[0145] Specifically, the system includes a processor, a memory, a bus, and a communication device; the memory is used to store computer-executable instructions, the processor is connected to the memory through the bus, and the processor executes the instructions stored in the...
PUM
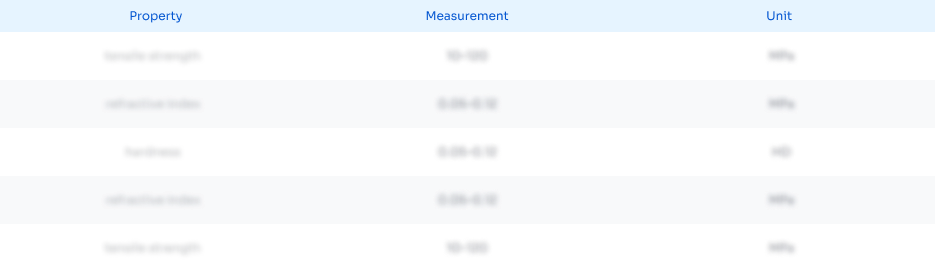
Abstract
Description
Claims
Application Information
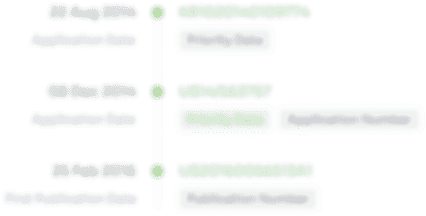
- R&D Engineer
- R&D Manager
- IP Professional
- Industry Leading Data Capabilities
- Powerful AI technology
- Patent DNA Extraction
Browse by: Latest US Patents, China's latest patents, Technical Efficacy Thesaurus, Application Domain, Technology Topic, Popular Technical Reports.
© 2024 PatSnap. All rights reserved.Legal|Privacy policy|Modern Slavery Act Transparency Statement|Sitemap|About US| Contact US: help@patsnap.com