Unsupervised target detection method and system based on variational auto-encoder and Gaussian mixture model
A Gaussian mixture model and target detection technology, applied in the field of artificial intelligence, can solve the problems of insensitive object type information and insufficient detection accuracy, and achieve the effect of good performance and good effect.
- Summary
- Abstract
- Description
- Claims
- Application Information
AI Technical Summary
Problems solved by technology
Method used
Image
Examples
Embodiment Construction
[0014] Such as figure 1 As shown, this embodiment involves an unsupervised target detection system based on a variational autoencoder and a Gaussian mixture model, including: a backbone network and a pres-prediction head, a depth prediction head, and a where-prediction head respectively connected to it ; Spatial transformation network and what-encoder and cat-encoder respectively connected to it; cell decoder, what prior network and differentiable renderer, wherein: the backbone network preprocesses the input image to obtain the feature map, pres -prediction head, depth prediction head and where-prediction head respectively get z according to the feature map pres Hidden variable, z where Hidden variables and z depth Hidden variable, the spatial transformation network according to the input image and z where Hidden variables undergo spatial transformation processing and output cell information to cat-encoder and what-encoder respectively, and cat-encoder obtains z according ...
PUM
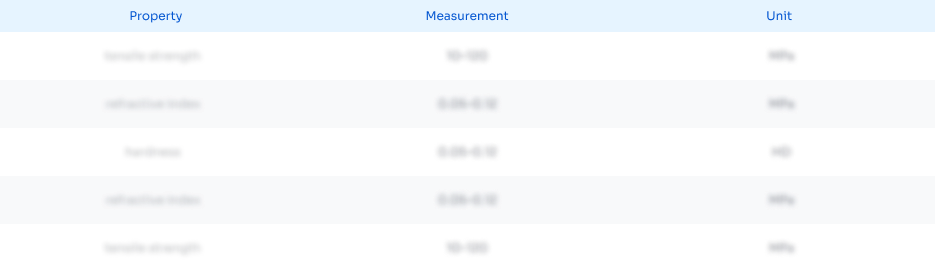
Abstract
Description
Claims
Application Information
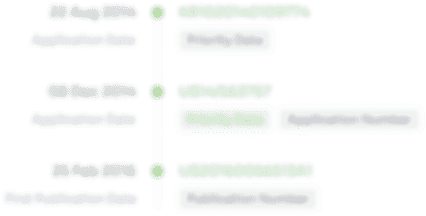
- R&D Engineer
- R&D Manager
- IP Professional
- Industry Leading Data Capabilities
- Powerful AI technology
- Patent DNA Extraction
Browse by: Latest US Patents, China's latest patents, Technical Efficacy Thesaurus, Application Domain, Technology Topic.
© 2024 PatSnap. All rights reserved.Legal|Privacy policy|Modern Slavery Act Transparency Statement|Sitemap