Image style migration method based on style and content decoupling
A content image and style technology, applied in the field of image style transfer based on the decoupling of style and content, can solve the problems of inability to extract feature information, unsatisfactory stylization effect, and style limitation, and achieve better rendering effect. Effect
- Summary
- Abstract
- Description
- Claims
- Application Information
AI Technical Summary
Problems solved by technology
Method used
Image
Examples
Embodiment Construction
[0024] Specific embodiments of the present invention will be described in detail below in conjunction with the accompanying drawings.
[0025] Such as figure 1 As shown, the present invention proposes an image style transfer method based on the decoupling of style and content, and the specific steps are as follows:
[0026] Step 1, model design.
[0027] The model of the invention consists of two parts: an image style conversion neural network and a loss measurement network based on the decoupling of style and content.
[0028] The image style conversion network of the present invention belongs to a residual network, and the network is composed of 3 convolutional layers, 5 residual blocks, and 3 upsampling convolutional layers.
[0029] The loss measurement network based on the decoupling of style and content in the present invention includes a style feature extraction module and a content feature extraction module. Among them, the content feature extraction module adopts t...
PUM
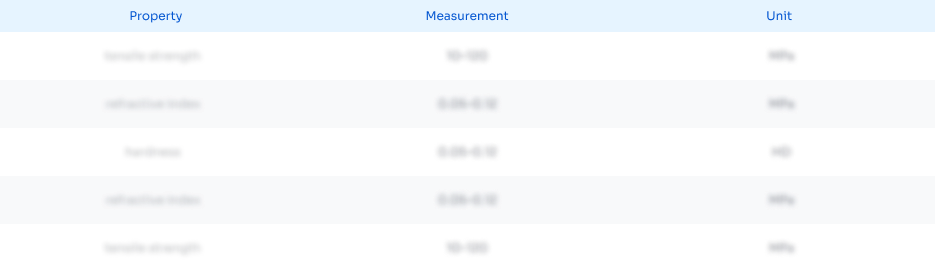
Abstract
Description
Claims
Application Information
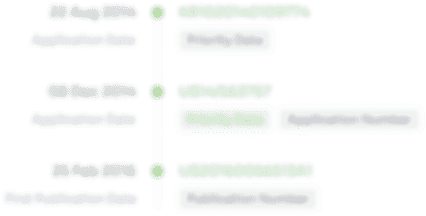
- R&D Engineer
- R&D Manager
- IP Professional
- Industry Leading Data Capabilities
- Powerful AI technology
- Patent DNA Extraction
Browse by: Latest US Patents, China's latest patents, Technical Efficacy Thesaurus, Application Domain, Technology Topic, Popular Technical Reports.
© 2024 PatSnap. All rights reserved.Legal|Privacy policy|Modern Slavery Act Transparency Statement|Sitemap|About US| Contact US: help@patsnap.com