A gradient perturbation-based federated learning data privacy protection method and system
A data privacy and gradient technology, applied in the direction of digital data protection, integrated learning, electronic digital data processing, etc., can solve the problems of reducing model availability, defense scheme noise disturbance, etc., to ensure classification accuracy, ensure that it is not leaked, and maintain The effect of usability
- Summary
- Abstract
- Description
- Claims
- Application Information
AI Technical Summary
Problems solved by technology
Method used
Image
Examples
Embodiment Construction
[0028] In order to make the objectives, technical solutions and advantages of the present invention, the present invention will be described in further detail below with reference to the accompanying drawings and examples. It should be understood that the specific embodiments described herein are merely intended to illustrate the invention and are not intended to limit the invention. Further, the technical features according to each of the various embodiments described below can be combined with each other as long as they do not constitute a collision between each other.
[0029] In the present invention, the terms "first", "second", or the like (if present), and the like in the drawings are used to distinguish the similar object without having to describe a particular order or ahead order.
[0030] figure 1 A flow chart of a gradient disturbance-based federal learning data privacy method is provided for the embodiment of the present invention. See figure 1 Combine figure 2 Furth...
PUM
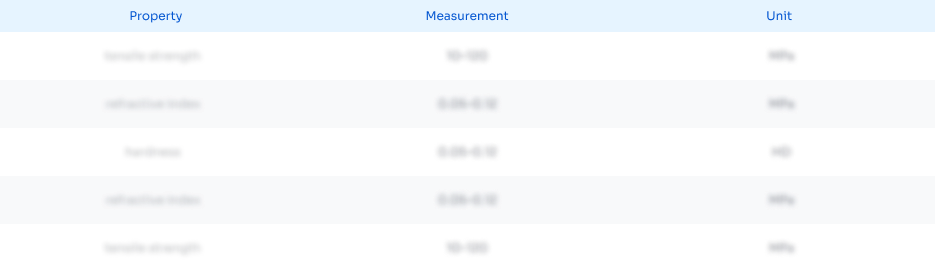
Abstract
Description
Claims
Application Information
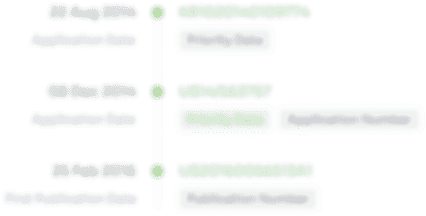
- R&D Engineer
- R&D Manager
- IP Professional
- Industry Leading Data Capabilities
- Powerful AI technology
- Patent DNA Extraction
Browse by: Latest US Patents, China's latest patents, Technical Efficacy Thesaurus, Application Domain, Technology Topic.
© 2024 PatSnap. All rights reserved.Legal|Privacy policy|Modern Slavery Act Transparency Statement|Sitemap