Medical image segmentation method and system based on AS-UNet
A medical image and image technology, applied in image analysis, neural learning methods, image enhancement, etc., can solve problems affecting medical judgment, parameter redundancy, unfavorable operation, etc., to improve segmentation ability, reduce time cost, and improve segmentation accuracy Effect
- Summary
- Abstract
- Description
- Claims
- Application Information
AI Technical Summary
Problems solved by technology
Method used
Image
Examples
Embodiment Construction
[0051] The present invention is further analyzed below in conjunction with accompanying drawing.
[0052] A medical image segmentation method based on AS-UNet comprises the following steps:
[0053] Step (1), obtain the original medical image to be segmented, preprocess it, and use the segmented medical image as a label to construct a training data set;
[0054] The preprocessing is to convert the original medical image into a fixed size of 512*512, and perform contrast enhancement processing. After the color image is grayscaled, the grayscale pixels are converted to between 0 and 1 to reduce the scale of the input feature.
[0055] Step (2), the label image in the training data set is processed by the mask edge extraction algorithm to obtain the mask edge image, image 3 Label image Mask and mask edge image Mask Boundary Image;
[0056] specifically is:
[0057] Copy the label image, and set all pixel values of the image to 255 to obtain a copy of the label image;
[00...
PUM
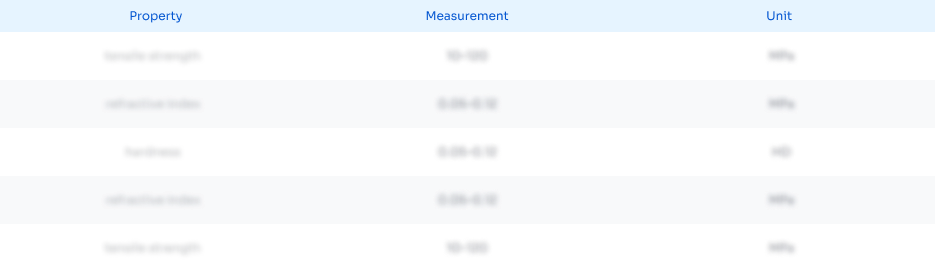
Abstract
Description
Claims
Application Information
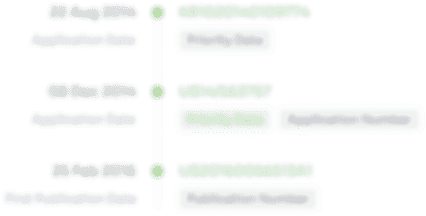
- R&D Engineer
- R&D Manager
- IP Professional
- Industry Leading Data Capabilities
- Powerful AI technology
- Patent DNA Extraction
Browse by: Latest US Patents, China's latest patents, Technical Efficacy Thesaurus, Application Domain, Technology Topic, Popular Technical Reports.
© 2024 PatSnap. All rights reserved.Legal|Privacy policy|Modern Slavery Act Transparency Statement|Sitemap|About US| Contact US: help@patsnap.com