Public digital life scene rule model prediction and early warning method based on deep Bayesian network
A Bayesian network and digital life technology, applied in the field of big data analysis, can solve problems such as low prediction accuracy and insufficient analysis features
- Summary
- Abstract
- Description
- Claims
- Application Information
AI Technical Summary
Problems solved by technology
Method used
Image
Examples
Embodiment 1
[0058] Example 1 - public health safety early warning
[0059] 1.1 Technical route
[0060] The traditional risk prediction of infectious disease outbreak mainly includes the following four aspects: (1) select the type of infectious disease and the research area; (2) select the pathological, environmental and climatic factors related to the incidence of infectious disease; (3) select the appropriate model to Establish a risk assessment model for infectious disease outbreaks; (4) predict the probability of infectious disease outbreaks in various situations and verify the accuracy of the model established. This example has made appropriate modifications on this basis. The specific technical route is as follows: Figure 8 shown.
[0061] Among them, the exploration of the spatio-temporal law of infectious diseases mainly uses statistics, mathematics and GIS methods. In this example, the detection of spatial clustering of infectious diseases is mainly reflected in the clustering...
Embodiment 2
[0135] Example 2—Mental Health Early Warning
[0136] The form of online questionnaire can effectively screen students for depression, and the online health questionnaire-depression scale (PHQ-9) can be used to collect students' self-assessment data online, but it is time-consuming and laborious, lacking real-time and reliability, The quality and quantity of data collected was low. Research by psychologists has shown that real-time screening of depression using data from social media such as WeChat and Weibo is feasible and accurate.
[0137] Therefore, this example combines the characteristics of students and uses social media data to construct a student word cloud. On this basis, it combines card data, Internet data and mobile terminal data, access records, consumption records, video surveillance, GPS, campus wifi access logs and other data to obtain spatio-temporal information, analyze students' behavior trajectory, and construct student portraits and information behavior ...
Embodiment 3
[0165] Example 3 - Early warning of campus bullying incidents
[0166] According to methods 2.1 to 2.3, the behavioral elements and information elements of past campus bullying incidents were obtained and analyzed, combined with student information, their personality, consumption situation, behavior habits, learning status, psychological status, etc. were analyzed, and user portraits of bullying students were constructed.
[0167] Refer to the method of risk probability estimation in 1.4 to construct a deep Bayesian network, construct a feature vector according to the characteristics of student user portraits and their behavior elements and information elements, and train to obtain a campus bullying early warning model; if the risk value exceeds 0.5, an alarm will be issued, and relevant students will be given corresponding measures Pay attention, and if necessary, provide psychological counseling, home visits or punishment.
PUM
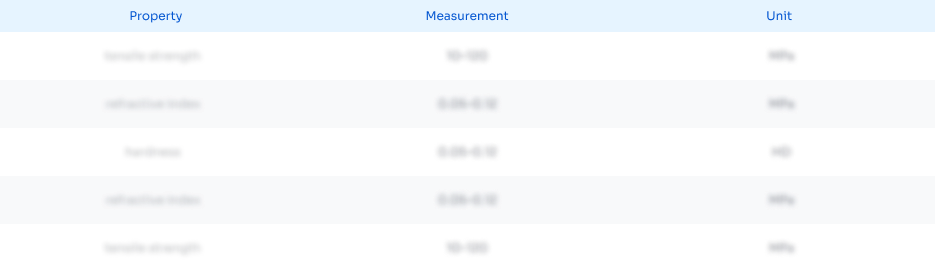
Abstract
Description
Claims
Application Information
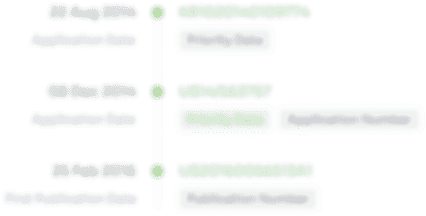
- R&D Engineer
- R&D Manager
- IP Professional
- Industry Leading Data Capabilities
- Powerful AI technology
- Patent DNA Extraction
Browse by: Latest US Patents, China's latest patents, Technical Efficacy Thesaurus, Application Domain, Technology Topic, Popular Technical Reports.
© 2024 PatSnap. All rights reserved.Legal|Privacy policy|Modern Slavery Act Transparency Statement|Sitemap|About US| Contact US: help@patsnap.com