Two-phase flow pattern identification method based on multi-scale convolutional network
A convolutional network and flow pattern recognition technology, applied in the field of image processing and deep learning, can solve problems such as insufficient image feature extraction, many layers of VGG model, and poor network generalization performance
- Summary
- Abstract
- Description
- Claims
- Application Information
AI Technical Summary
Problems solved by technology
Method used
Image
Examples
Embodiment Construction
[0044] The specific implementation steps of the present invention are as follows: a method for identifying two-phase flow patterns based on multi-scale convolutional networks, the frame diagram of which is as follows figure 1 shown, including the following steps:
[0045] Step 1: Use the RBF neural network for image reconstruction, construct the image data set of the convolutional neural network, that is, construct the image data set for two-phase flow flow pattern recognition, and classify the collected images into circular flow Type and core flow type, and divided into training set, verification set and test set according to the ratio of 4:1:1.
[0046] The specific operation is to build a 16-electrode ERT two-phase flow model through Comsol simulation software, collect boundary potential data as training samples and input them into the RBF neural network model for training, and finally input the test samples into the trained model and perform image reconstruction , by adju...
PUM
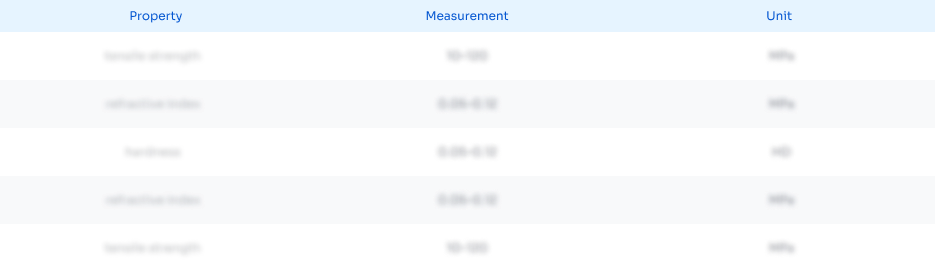
Abstract
Description
Claims
Application Information
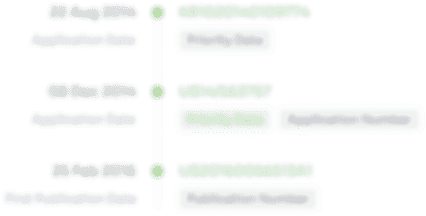
- R&D Engineer
- R&D Manager
- IP Professional
- Industry Leading Data Capabilities
- Powerful AI technology
- Patent DNA Extraction
Browse by: Latest US Patents, China's latest patents, Technical Efficacy Thesaurus, Application Domain, Technology Topic.
© 2024 PatSnap. All rights reserved.Legal|Privacy policy|Modern Slavery Act Transparency Statement|Sitemap