Non-invasive load identification method based on V-I trajectory diagram and neural network
A neural network and load recognition technology, applied in neural learning methods, biological neural network models, neural architectures, etc., can solve the problems of insufficient utilization of load power characteristics and V-I trajectory characteristics, and achieve good identification results.
- Summary
- Abstract
- Description
- Claims
- Application Information
AI Technical Summary
Problems solved by technology
Method used
Image
Examples
Embodiment Construction
[0031] The present invention is explained in conjunction with the accompanying drawings and the implementation of using the BLUED public data set. The specific implementation steps are as follows:
[0032] The invention provides a non-invasive load recognition method based on V-I trajectory diagram and neural network, such as figure 1 As shown, its implementation steps include:
[0033] S1: First extract 5 types of household electrical equipment from the BLUED dataset, then construct an RGB color map based on V-I trajectory features and train a convolutional neural network model as a recognition network, such as the Alexnet model. The recognition network model in this embodiment is shown in the figure. In order to be able to run directly on the MCU above STM32F7, the recognition network model built is not complicated, including two convolutional layers, two pooling layers and three full layers. The connection layer, the specific structure is as figure 2 shown.
[0034] S2:...
PUM
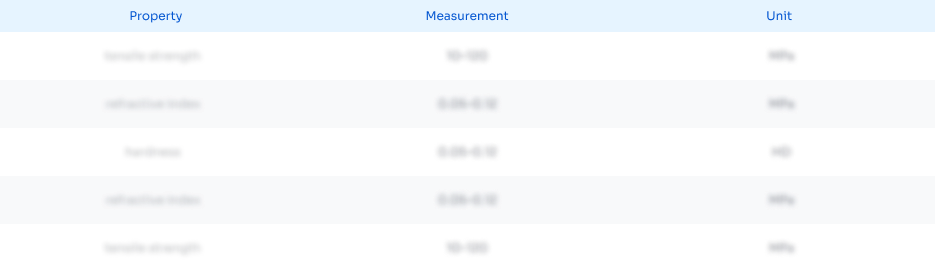
Abstract
Description
Claims
Application Information
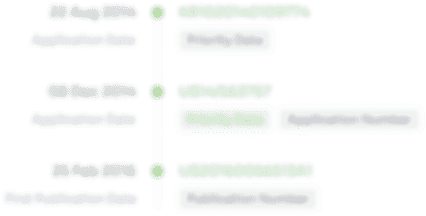
- R&D Engineer
- R&D Manager
- IP Professional
- Industry Leading Data Capabilities
- Powerful AI technology
- Patent DNA Extraction
Browse by: Latest US Patents, China's latest patents, Technical Efficacy Thesaurus, Application Domain, Technology Topic, Popular Technical Reports.
© 2024 PatSnap. All rights reserved.Legal|Privacy policy|Modern Slavery Act Transparency Statement|Sitemap|About US| Contact US: help@patsnap.com