Machine learning estimation method for sensitivity and mechanical properties of energetic substances and relationship between the sensitivity and the mechanical properties of the energetic substances
A machine learning and material technology, applied in chemical machine learning, chemical statistics, chemical property prediction, etc., can solve problems such as time-consuming, inability to calculate a large number of samples in a short time, and greatly improving prediction accuracy.
- Summary
- Abstract
- Description
- Claims
- Application Information
AI Technical Summary
Problems solved by technology
Method used
Image
Examples
Embodiment 1
[0140] 1. Introduction
[0141] The present invention intends to adopt two methods of ANN and SISSO to study the relationship between the impact sensitivity and mechanical properties of nitro energetic substances, as well as the quantitative relationship between the two and the molecular structure. The research content includes the following four parts.
[0142] 1) Establish the QSPR model of the mechanical properties and molecular structure of energetic materials. Nitro compounds, as a high-energy-density material (HEDM) widely used in civilian and military applications, are still the most dominant and important part of explosives today. Almost all energetic materials contain nitro groups (X–NO 2 , X=C, N or O), the nitro group provides nitrogen element for the energetic molecule, which can ensure its decomposition into N 2 When releasing a large amount of energy, it also provides the essential oxygen element in the combustion or detonation process of high-energy materials...
PUM
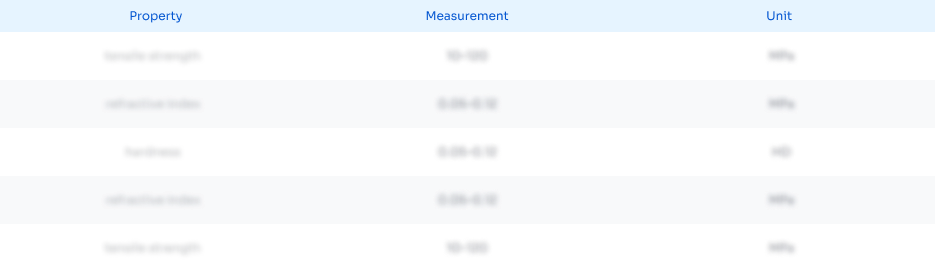
Abstract
Description
Claims
Application Information
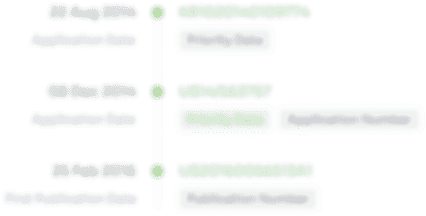
- R&D Engineer
- R&D Manager
- IP Professional
- Industry Leading Data Capabilities
- Powerful AI technology
- Patent DNA Extraction
Browse by: Latest US Patents, China's latest patents, Technical Efficacy Thesaurus, Application Domain, Technology Topic, Popular Technical Reports.
© 2024 PatSnap. All rights reserved.Legal|Privacy policy|Modern Slavery Act Transparency Statement|Sitemap|About US| Contact US: help@patsnap.com