Mechanical fault diagnosis method based on multi-sensor information fusion migration network
A multi-sensor, mechanical failure technology, applied in the testing of mechanical components, biological neural network models, and testing of machine/structural components, etc., can solve the problems of unsatisfactory fusion effect and inability to automatically learn deep feature representation.
- Summary
- Abstract
- Description
- Claims
- Application Information
AI Technical Summary
Problems solved by technology
Method used
Image
Examples
Embodiment 1
[0081] This embodiment discloses a mechanical fault diagnosis method based on a multi-sensor information fusion migration network. The method uses a feature sharing layer and a plurality of convolutional neural networks to perform a diagnosis based on the historical data of multiple source domain sensors in the source domain diagnosis task. learning, and transfer the knowledge learned on the labeled source domain dataset to the unlabeled target domain task, improving the diagnostic performance of the target domain task. like figure 1 As shown, the steps are as follows:
[0082] S1. Multi-sensor data acquisition: According to the actual operating conditions of mechanical equipment, arrange source domain sensors for collecting fault data at different positions of mechanical equipment, and then use source domain sensors to obtain fault data with different sensitivities to faults at each position, Thereby forming multiple source domain datasets Among them, the source domain datas...
Embodiment 2
[0136] This embodiment discloses a mechanical fault diagnosis device based on multi-sensor information fusion migration network, such as Figure 5 shown, including:
[0137] The source domain sensor data acquisition module is used to use the source domain sensors arranged at different positions of the mechanical equipment according to the actual operating conditions of the mechanical equipment to obtain fault data with different sensitivities to faults at each position, thereby forming multiple source domain data sets Among them, the source domain dataset consists of source domain sensor data x s and its corresponding label y s Composition, the label corresponds to the health status of the source domain diagnosis task, M represents the number of source domain sensors, and also represents the number of source domain data sets, j represents the serial number of the source domain sensor, and also represents the serial number of the source domain data set;
[0138] The target d...
Embodiment 3
[0159] This embodiment discloses a storage medium, which stores a program. When the program is executed by a processor, the method for diagnosing mechanical faults based on the multi-sensor information fusion migration network described in Embodiment 1 is implemented, specifically as follows:
[0160] S1. Multi-sensor data acquisition: According to the actual operating conditions of mechanical equipment, arrange source domain sensors for collecting fault data at different positions of mechanical equipment, and then use source domain sensors to obtain fault data with different sensitivities to faults at each position, Thereby forming multiple source domain datasets Among them, the source domain dataset consists of source domain sensor data x s and its corresponding label y s Composition, the label corresponds to the health status of the source domain diagnosis task, M represents the number of source domain sensors, and also represents the number of source domain data sets, j ...
PUM
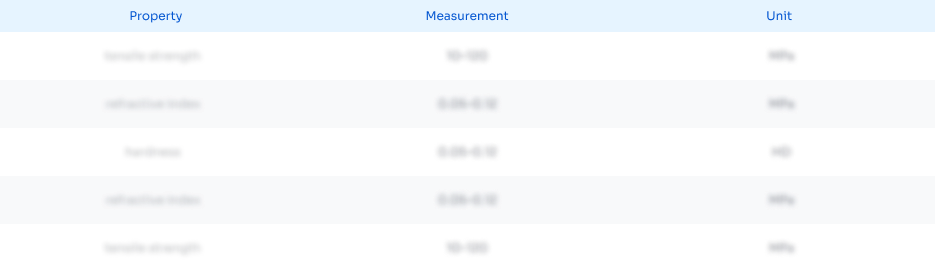
Abstract
Description
Claims
Application Information
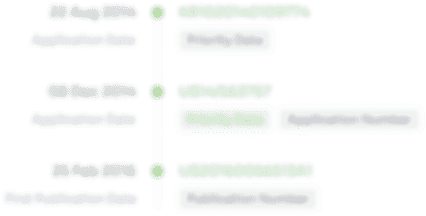
- Generate Ideas
- Intellectual Property
- Life Sciences
- Materials
- Tech Scout
- Unparalleled Data Quality
- Higher Quality Content
- 60% Fewer Hallucinations
Browse by: Latest US Patents, China's latest patents, Technical Efficacy Thesaurus, Application Domain, Technology Topic, Popular Technical Reports.
© 2025 PatSnap. All rights reserved.Legal|Privacy policy|Modern Slavery Act Transparency Statement|Sitemap|About US| Contact US: help@patsnap.com