Low-latency collaborative adaptive CNN inference system and low-latency collaborative adaptive CNN inference method
An adaptive, low-latency technology, applied in the field of edge computing and deep learning, which can solve the problems of high synchronization overhead, fixed scheduling strategy, and speeding up CNN inference speed.
- Summary
- Abstract
- Description
- Claims
- Application Information
AI Technical Summary
Problems solved by technology
Method used
Image
Examples
Embodiment Construction
[0064] The technical solution of the present invention will be further described below in conjunction with the accompanying drawings.
[0065] According to an embodiment of the present invention, a distributed CNN inference system for CNN tasks under the edge network is a collaborative system composed of a single master node and multiple slave nodes, wherein the master node monitors the running status of each slave node in real time, And periodically update slave node tasks to coordinate global tasks. There is only one master node in the system; slave nodes are responsible for inferring the specific execution of tasks, and there are usually multiple slave nodes. Both the master node and the slave node maintain the structural information of CNN, and use the cross-layer data range deduction mechanism to calculate the dependency between any two pieces of data.
[0066] Specifically, refer tofigure 1 , the architecture of the master node on the right, including: running informatio...
PUM
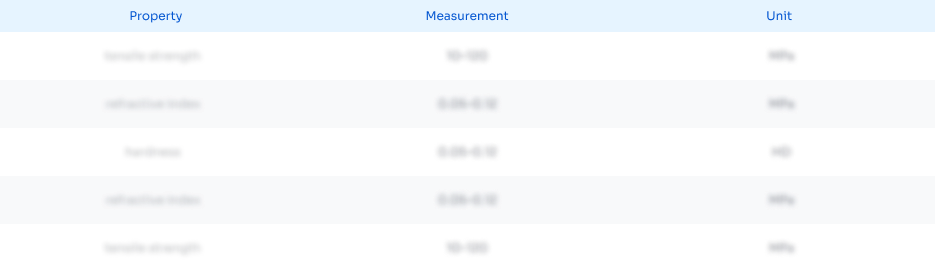
Abstract
Description
Claims
Application Information
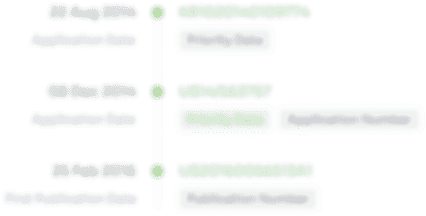
- R&D Engineer
- R&D Manager
- IP Professional
- Industry Leading Data Capabilities
- Powerful AI technology
- Patent DNA Extraction
Browse by: Latest US Patents, China's latest patents, Technical Efficacy Thesaurus, Application Domain, Technology Topic, Popular Technical Reports.
© 2024 PatSnap. All rights reserved.Legal|Privacy policy|Modern Slavery Act Transparency Statement|Sitemap|About US| Contact US: help@patsnap.com