Urban noise identification method of multilayer random neural network based on quantization error entropy
A stochastic neural network and quantization error technology, applied in biological neural network models, speech recognition, neural architecture, etc., can solve problems such as rarely considering the existence of acquisition equipment noise, and difficult to achieve ideal classification results
- Summary
- Abstract
- Description
- Claims
- Application Information
AI Technical Summary
Problems solved by technology
Method used
Image
Examples
specific Embodiment approach
[0094] Such as Figure 1-4 Shown, the first main step of the present invention, its step is as follows:
[0095] 1-1. Collect different sound signals and build a sound database.
[0096] 1-2. Pre-emphasize the original sound data.
[0097] 1-3. Framing the data.
[0098] 1-4. Perform windowing processing on the data.
[0099] 1-5. Perform fast Fourier transform on the data.
[0100] 1-6. Pass the data through a triangular bandpass filter.
[0101] 1-7. Calculate the logarithmic energy after passing through the filter, and then enter the discrete cosine transform to obtain the MFCC coefficient.
[0102] The second main step of the present invention is to the quantization of error, and its step is as follows:
[0103] 2-1. Set the quantization threshold ε, and initialize the quantization code table.
[0104] 2-1. Calculate the distance between an error and each value in the code table, and take the minimum value. If the value is less than the quantization threshold, keep t...
PUM
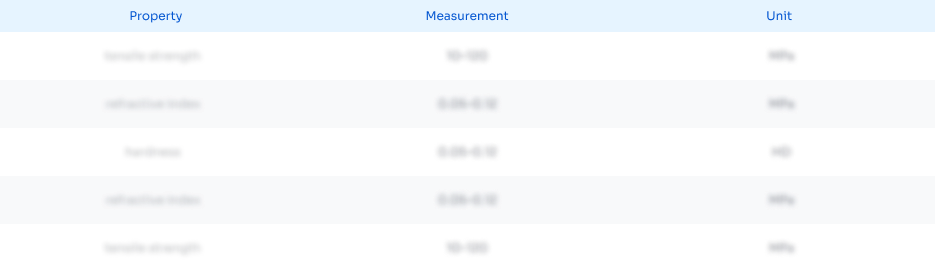
Abstract
Description
Claims
Application Information
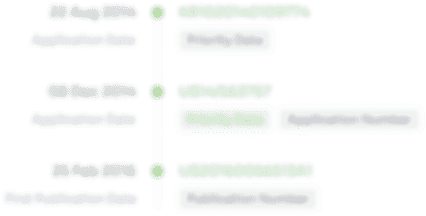
- Generate Ideas
- Intellectual Property
- Life Sciences
- Materials
- Tech Scout
- Unparalleled Data Quality
- Higher Quality Content
- 60% Fewer Hallucinations
Browse by: Latest US Patents, China's latest patents, Technical Efficacy Thesaurus, Application Domain, Technology Topic, Popular Technical Reports.
© 2025 PatSnap. All rights reserved.Legal|Privacy policy|Modern Slavery Act Transparency Statement|Sitemap|About US| Contact US: help@patsnap.com