Entity identification method and device, electronic equipment and storage medium
A technology for entity recognition and entity grouping, applied in neural learning methods, electrical digital data processing, instruments, etc., can solve problems such as poor portability, cumbersome feature selection, and a large number of problems, and achieve the effect of improving representation ability and high labeling efficiency
- Summary
- Abstract
- Description
- Claims
- Application Information
AI Technical Summary
Problems solved by technology
Method used
Image
Examples
Embodiment 1
[0048] Embodiment 1 provides an entity recognition method, please refer to figure 1 shown, including the following steps:
[0049] S110, learn the text to be marked based on the BERT model to obtain word vectors of each word in the text to be marked, and form a text vector from the word vectors of each word.
[0050] The BERT (Bidirectional Encoder Representations from Transformers) model is a deep bidirectional pre-trained language understanding model using the Transformer model as a feature extractor. Essentially, it learns a good feature representation for words by running a self-supervised learning method on the basis of massive corpus. , self-supervised learning refers to supervised learning that operates on data that has not been manually labeled. The Transformer model is a classic NLP model proposed by the Google team. For example, the following formula models a piece of text based on the attention mechanism, which can be trained in parallel and has global information....
Embodiment 2
[0075] Embodiment 2 is an improvement on the basis of Embodiment 1, please refer to figure 2 As shown, the text to be marked is learned based on the BERT model to obtain the word vector of each word in the text to be marked, and the word vector of each word is used to form a text vector, including the following steps:
[0076] S210. Place a sentence start tag, a sentence end tag, and a segmentation tag respectively at the beginning of a sentence, at the end of a sentence of the text to be tagged, and between two sentences in the text to be tagged to obtain an intermediate text. Usually, the sentence beginning label, sentence end label and segmentation label use the [CLS] label, [SEP] label and [SEP] label respectively, and it is convenient to obtain the context information of each word in the text to be labeled when learning based on the BERT model .
[0077] S220. Segment the intermediate text at the character level to obtain a plurality of words, randomly select several wo...
Embodiment 3
[0093] Embodiment 3 discloses an entity recognition device corresponding to the above embodiment, which is the virtual device structure of the above embodiment, please refer to image 3 shown, including:
[0094] The text vector calculation module 410 is used to learn the text to be marked based on the BERT model to obtain the word vector of each word in the text to be marked, and form the text vector by the word vector of each of the words;
[0095] A model set and an unlabeled corpus acquisition module 420, configured to acquire a model set including N preliminary trained neural network models and an unlabeled corpus including a plurality of unlabeled texts, and convert the N preliminary trained neural networks The models are respectively recorded as mi, i=1,..., N, N>2;
[0096] The collaborative training module 430 is configured to, for each of the initially trained neural network models mi, identify each of the unlabeled texts based on other N-1 initially trained neural ...
PUM
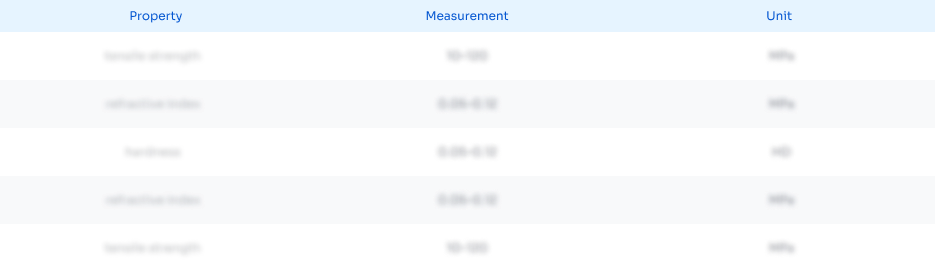
Abstract
Description
Claims
Application Information
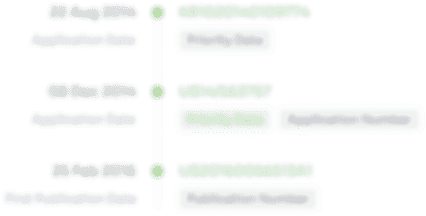
- R&D Engineer
- R&D Manager
- IP Professional
- Industry Leading Data Capabilities
- Powerful AI technology
- Patent DNA Extraction
Browse by: Latest US Patents, China's latest patents, Technical Efficacy Thesaurus, Application Domain, Technology Topic, Popular Technical Reports.
© 2024 PatSnap. All rights reserved.Legal|Privacy policy|Modern Slavery Act Transparency Statement|Sitemap|About US| Contact US: help@patsnap.com