Adversarial sample generation method based on content-aware GAN
A technology of adversarial samples and content perception, applied in the field of deep learning, can solve problems such as low quality of adversarial samples, low attack success rate, and easy identification of disturbances, so as to reduce the degree of perceptibility, increase the attack success rate, and improve speed effect
- Summary
- Abstract
- Description
- Claims
- Application Information
AI Technical Summary
Problems solved by technology
Method used
Image
Examples
Embodiment Construction
[0033] In order to make the above-mentioned features and advantages of the present invention more comprehensible, the present invention will be further described in detail below in conjunction with specific embodiments and accompanying drawings.
[0034] The adversarial sample generation method designed by the present invention is based on the basic attack method WGAN_GP. By using the unsupervised training phase of two different targets, the loss function of the model adversarial training phase is designed, so that the GAN model can learn the distribution of adversarial samples from random noise, Generate unlimited adversarial samples in batches to conduct adversarial attacks on the target model. The specific training process is as figure 1 As shown, its main steps include:
[0035] Step 100, training WGAN_GP to learn the data distribution of normal samples, the structure diagram of the normal training stage is as follows figure 2 shown.
[0036] Further, step 100 specific...
PUM
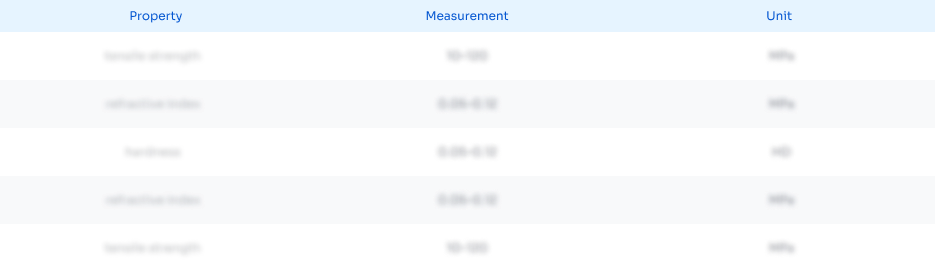
Abstract
Description
Claims
Application Information
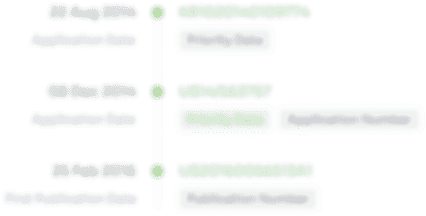
- R&D Engineer
- R&D Manager
- IP Professional
- Industry Leading Data Capabilities
- Powerful AI technology
- Patent DNA Extraction
Browse by: Latest US Patents, China's latest patents, Technical Efficacy Thesaurus, Application Domain, Technology Topic, Popular Technical Reports.
© 2024 PatSnap. All rights reserved.Legal|Privacy policy|Modern Slavery Act Transparency Statement|Sitemap|About US| Contact US: help@patsnap.com