Human body activity recognition method based on grouping residual joint spatial learning
A human activity and residual technology, applied in neural learning methods, character and pattern recognition, instruments, etc., can solve problems such as low accuracy, and achieve the effect of improving accuracy, increasing inter-class distance, and reducing intra-class distance
- Summary
- Abstract
- Description
- Claims
- Application Information
AI Technical Summary
Problems solved by technology
Method used
Image
Examples
Embodiment Construction
[0023] The present invention will be further described below in conjunction with the accompanying drawings.
[0024] refer to figure 1 and figure 2 , a human activity recognition method based on joint spatial learning of group residuals, including the following steps:
[0025] Step 1. Group, align, and slice single-channel data based on the sliding window. The process is as follows:
[0026] Step 1.1: Collect human activities through wearable sensors, object sensors, and environmental sensors to generate a subset of gesture activity category data, and combine various human activity signals to construct a human activity recognition data set;
[0027] Step 1.2: Use the sliding window method to group, align and slice the data, convert the serialized data into single-channel two-dimensional data, and use the two-dimensional data to extract high-level abstract semantic features;
[0028] Step 1.3: Classify the two-dimensional data subset according to the gesture activity catego...
PUM
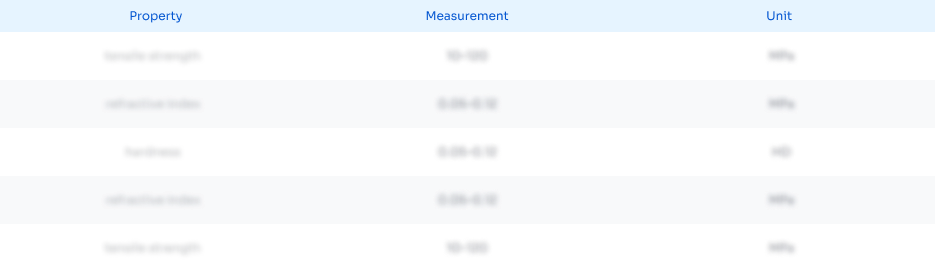
Abstract
Description
Claims
Application Information
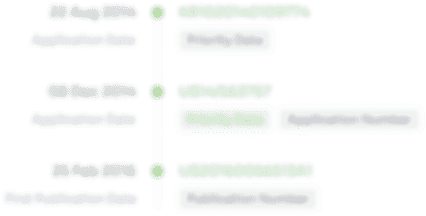
- R&D Engineer
- R&D Manager
- IP Professional
- Industry Leading Data Capabilities
- Powerful AI technology
- Patent DNA Extraction
Browse by: Latest US Patents, China's latest patents, Technical Efficacy Thesaurus, Application Domain, Technology Topic, Popular Technical Reports.
© 2024 PatSnap. All rights reserved.Legal|Privacy policy|Modern Slavery Act Transparency Statement|Sitemap|About US| Contact US: help@patsnap.com