Semantic SLAM robustness improvement method based on instance segmentation
A robust and semantic technology, applied in the field of dynamic scene semantic SLAM improvement, can solve the problems of low accuracy and large amount of calculation
- Summary
- Abstract
- Description
- Claims
- Application Information
AI Technical Summary
Problems solved by technology
Method used
Image
Examples
Embodiment
[0052] A method for improving robustness of semantic SLAM based on instance segmentation provided by an embodiment of the present invention includes the following steps:
[0053] (1) Use the RGB-D camera to obtain the RGB color image and the depth image, and the whole method uses three branches for parallel processing. Use branch three to process the depth image, and branch one and branch two to process the RGB color image, in which branch one divides the collected RGB color image data samples into training set, verification set and The test set, Branch 2 uses all RGB color image data for ORB feature extraction and assists optical flow calculation;
[0054] (2) In branch one, for the processing of the RGB color image, the training set after the division of the RGB color image is sent to the instance segmentation convolutional neural network training and the final network model parameters are obtained after verifying the effect on the verification set. The instance segmentatio...
PUM
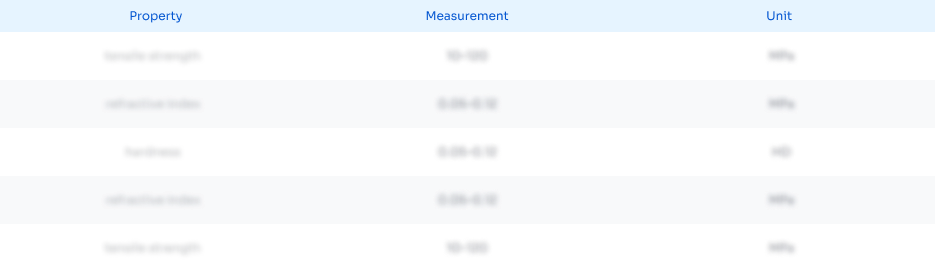
Abstract
Description
Claims
Application Information
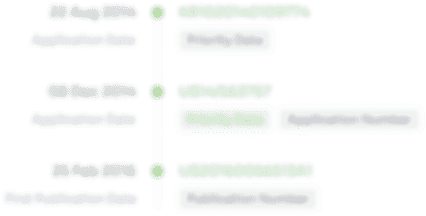
- R&D Engineer
- R&D Manager
- IP Professional
- Industry Leading Data Capabilities
- Powerful AI technology
- Patent DNA Extraction
Browse by: Latest US Patents, China's latest patents, Technical Efficacy Thesaurus, Application Domain, Technology Topic, Popular Technical Reports.
© 2024 PatSnap. All rights reserved.Legal|Privacy policy|Modern Slavery Act Transparency Statement|Sitemap|About US| Contact US: help@patsnap.com