Interpretable CNN image classification model-based optical remote sensing image classification method
A classification model and optical remote sensing technology, applied in the field of image processing, can solve the problems of low efficiency in the training process and low accuracy of image classification, and achieve the effects of enhancing interpretability, improving classification accuracy, and reducing time-consuming
- Summary
- Abstract
- Description
- Claims
- Application Information
AI Technical Summary
Problems solved by technology
Method used
Image
Examples
Embodiment Construction
[0028] The invention provides a method for classifying optical remote sensing images based on an interpretable CNN image classification model, by building an interpretable CNN network and selecting training samples and test samples; retraining the convolutional neural network ResNet model; performing an interpretability test; using The trained model is finally tested on the test set. The invention can quickly achieve the required recognition rate, reduces the time consumption of the network training process, improves the accuracy rate of remote sensing image classification, and improves the interpretability of the neural network model.
[0029] see figure 1 , an optical remote sensing image classification method based on an interpretable CNN image classification model in the present invention, whether 34 should be deleted. In the process of classifying optical remote sensing images in the general convolutional neural network, the features obtained by downsampling lose a lot o...
PUM
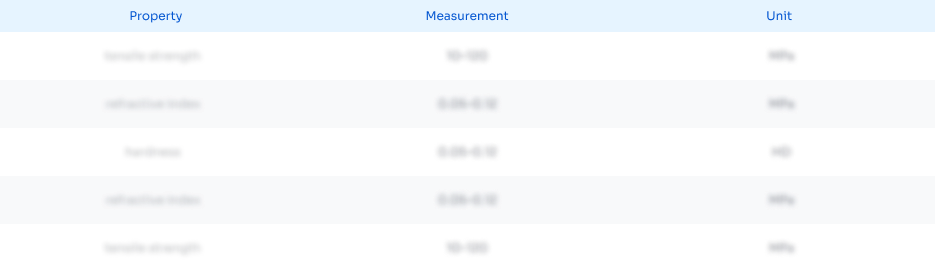
Abstract
Description
Claims
Application Information
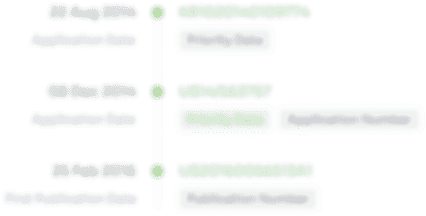
- R&D Engineer
- R&D Manager
- IP Professional
- Industry Leading Data Capabilities
- Powerful AI technology
- Patent DNA Extraction
Browse by: Latest US Patents, China's latest patents, Technical Efficacy Thesaurus, Application Domain, Technology Topic, Popular Technical Reports.
© 2024 PatSnap. All rights reserved.Legal|Privacy policy|Modern Slavery Act Transparency Statement|Sitemap|About US| Contact US: help@patsnap.com