Deep learning adversarial sample generation method based on second-order method
An adversarial sample, deep learning technology, applied in neural learning methods, biological neural network models, neural architectures, etc., can solve problems such as the poor speed of adversarial sample generation methods, the inability to generate adversarial samples, and the difficulty of optimization problems.
- Summary
- Abstract
- Description
- Claims
- Application Information
AI Technical Summary
Problems solved by technology
Method used
Image
Examples
Embodiment 1
[0134] 1. The specific analysis of related technologies is as follows:
[0135] 1.1 Deep Neural Networks and Symbols
[0136] DNNs can generally be expressed as a mapping function is the d-dimensional input variable, is an m-dimensional probability vector, representing the confidence of m classes. An N-layer DNNs receives an input X and produces the corresponding output as follows:
[0137] F(X)=F (N) (...F (2) (F (1) (X))) (1)
[0138] f (i) Represents the calculation output of the i-th layer of DNNs. These layers can be convolutional, pooling, or other forms of neural network layers. The last layer of DNNs uses a Softmax layer, defined as Z=F (N-1) ( ) is the output vector of the previous layer (aka the last hidden layer). The final predicted label is determined by y=argmax i=1...m F(X) i Obtain, where F(X)=Softmax(Z).
[0139] 1.2 Adversarial Examples for DNNs
[0140] Existing Technique 1 first discovered the existence of adversarial examples in DNNs. ...
PUM
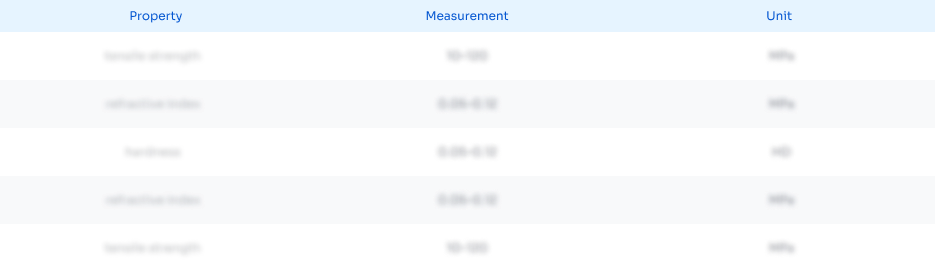
Abstract
Description
Claims
Application Information
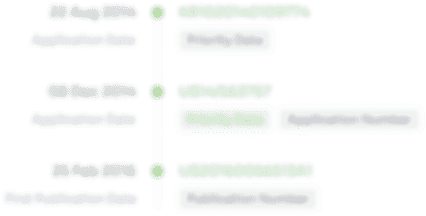
- R&D Engineer
- R&D Manager
- IP Professional
- Industry Leading Data Capabilities
- Powerful AI technology
- Patent DNA Extraction
Browse by: Latest US Patents, China's latest patents, Technical Efficacy Thesaurus, Application Domain, Technology Topic, Popular Technical Reports.
© 2024 PatSnap. All rights reserved.Legal|Privacy policy|Modern Slavery Act Transparency Statement|Sitemap|About US| Contact US: help@patsnap.com