Target detection method based on non-convex motion assistance
A target detection and moving target technology, applied in the field of computer vision, can solve the problem of low detection accuracy, achieve the effect of improving accuracy, superiority and effectiveness
- Summary
- Abstract
- Description
- Claims
- Application Information
AI Technical Summary
Problems solved by technology
Method used
Image
Examples
Embodiment Construction
[0061] The technical solution of the present invention will be further described in conjunction with the accompanying drawings and embodiments.
[0062] The present invention adopts the non-convex gamma norm to replace the rank function in the traditional low-rank sparse decomposition model to approximately represent the low-rank part of the video background, and considering that the background still has the characteristics of sparsity in the transform domain, adopt l 1 The norm performs sparse approximation on the background of the transform domain, and the foreground still uses the l which represents the sparse prior of the moving target. 1 norm. In addition, the motion auxiliary information matrix is introduced into the above model to integrate the motion information of the foreground, so as to better realize the detection of moving objects in the video. So the following Nonconvex Motion-Assisted LowRank and Sparse Decomposition (NMALRSD) model is proposed:
[0063] ...
PUM
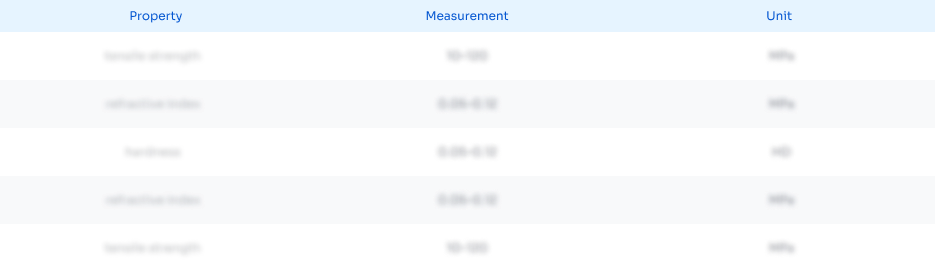
Abstract
Description
Claims
Application Information
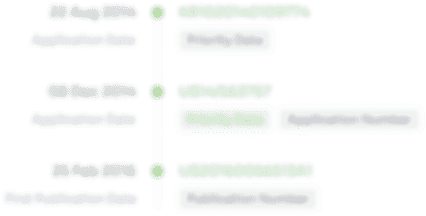
- R&D Engineer
- R&D Manager
- IP Professional
- Industry Leading Data Capabilities
- Powerful AI technology
- Patent DNA Extraction
Browse by: Latest US Patents, China's latest patents, Technical Efficacy Thesaurus, Application Domain, Technology Topic, Popular Technical Reports.
© 2024 PatSnap. All rights reserved.Legal|Privacy policy|Modern Slavery Act Transparency Statement|Sitemap|About US| Contact US: help@patsnap.com