Rolling bearing fault diagnosis method based on manifold learning and s-k-means clustering
A rolling bearing and manifold learning technology, applied in the testing of mechanical components, testing of machine/structural components, measuring devices, etc., can solve the problems of K-means clustering algorithm dependence, easy to be affected by isolated points, etc. The identification is convenient and effective, avoiding the disaster of dimensionality, and the effect of small calculation amount
- Summary
- Abstract
- Description
- Claims
- Application Information
AI Technical Summary
Problems solved by technology
Method used
Image
Examples
Embodiment Construction
[0058] The present invention is a rolling bearing fault diagnosis algorithm. The present invention will be further described in detail below in conjunction with the accompanying drawings.
[0059] Taking some experimental data from the bearing vibration database of Case Western Reserve University as an example, the bearings used are SKF 6205 single row deep groove ball bearings. For normal bearings, 0.007-inch single-point inner ring fault, 0.007-inch single-point outer ring fault, 0.007-inch single-point rolling element fault and 4 different degrees (0.007 inch, 0.014 inch, 0.021 inch, 0.028 inch) Single-point inner race failure (1 inch = 2.54 cm), analyzed.
[0060] The overall step flow chart of the rolling bearing fault diagnosis method disclosed in the present invention is as follows: figure 1 As shown, the specific steps are as follows:
[0061] 1. Obtain vibration acceleration signals of rolling bearings under different operating conditions, and obtain time-domain sig...
PUM
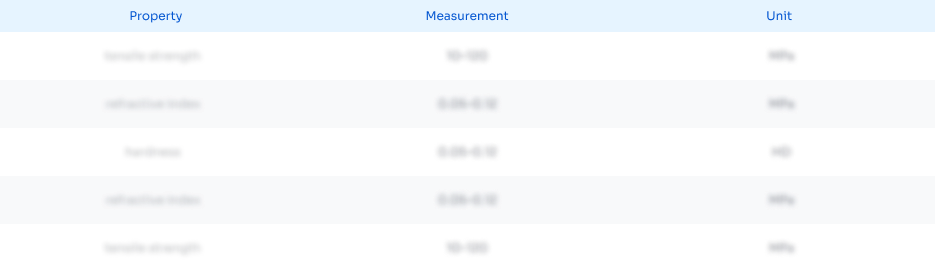
Abstract
Description
Claims
Application Information
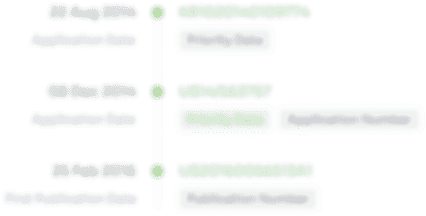
- R&D Engineer
- R&D Manager
- IP Professional
- Industry Leading Data Capabilities
- Powerful AI technology
- Patent DNA Extraction
Browse by: Latest US Patents, China's latest patents, Technical Efficacy Thesaurus, Application Domain, Technology Topic, Popular Technical Reports.
© 2024 PatSnap. All rights reserved.Legal|Privacy policy|Modern Slavery Act Transparency Statement|Sitemap|About US| Contact US: help@patsnap.com