Garbage classification method based on lightweight convolutional neural network
A convolutional neural network and garbage classification technology, applied in the field of garbage classification, can solve problems such as low model complexity and high classification accuracy at the same time, and achieve the effect of low complexity and high classification accuracy
- Summary
- Abstract
- Description
- Claims
- Application Information
AI Technical Summary
Problems solved by technology
Method used
Image
Examples
specific Embodiment approach 1
[0032] Specific implementation mode one: as figure 1 As shown, a kind of garbage classification method based on lightweight convolutional neural network described in this embodiment comprises the following steps:
[0033] Step 1. Obtain the original garbage image data set, and perform data enhancement on the acquired image to obtain the image data set after data enhancement;
[0034] Step 2, preprocessing the image data set after data enhancement to obtain the preprocessed image data set;
[0035]Step 3, constructing a convolutional neural network model, training the constructed convolutional neural network on the preprocessed image data set, and obtaining a trained convolutional neural network model;
[0036] The structure of the convolutional neural network model is: starting from the input end of the convolutional neural network model, the convolutional neural network model sequentially includes an input layer, a first sub-convolution unit, a second sub-convolution unit, a...
specific Embodiment approach 2
[0052] Specific embodiment 2: The difference between this embodiment and specific embodiment 1 is: in the step 1, data enhancement is performed on the acquired image to obtain a data-enhanced image data set. The specific process is as follows:
[0053] Carry out data enhancement on the acquired image, the method of data enhancement is: set the angle range of the random rotation of the image to 0°~20°, the offset coefficient of the image in the horizontal and vertical direction is 0.2, and the filling method is adjacent filling ;
[0054] After the data augmentation is completed, the image dataset after data augmentation is obtained.
[0055] The data set TrashNet used in the present invention only contains 2527 pieces of garbage images, including cardboard, glass, metal, paper, plastic, and 6 categories of garbage, with a total of 2527 pieces of RGB three-color images. Among them: 403 pieces of cardboard, 501 pieces of glass, 410 pieces of metal, 594 pieces of paper, 482 piec...
specific Embodiment approach 3
[0057] Embodiment 3: The difference between this embodiment and Embodiment 1 is that in the step 2, the image data set after data enhancement is preprocessed to obtain the preprocessed image data set. The specific process is as follows:
[0058] The size of each image in the image data set after data enhancement is uniformly scaled to the size of (224,224), and each value in the generated matrix is multiplied by 1 / 255, so that each value is between 0 and 1, Obtain the preprocessed image dataset.
[0059] For data with relatively large values or heterogeneous data (for example, one eigenvalue of the data is in the range of 0 to 1, and the other eigenvalue is in the range of 100 to 200), it is not appropriate to input it into the neural network, so Doing so may result in large gradient updates, which in turn will cause the network to fail to converge. In order to make the learning of the network easier, the input data should have the following characteristics: ①Small value:...
PUM
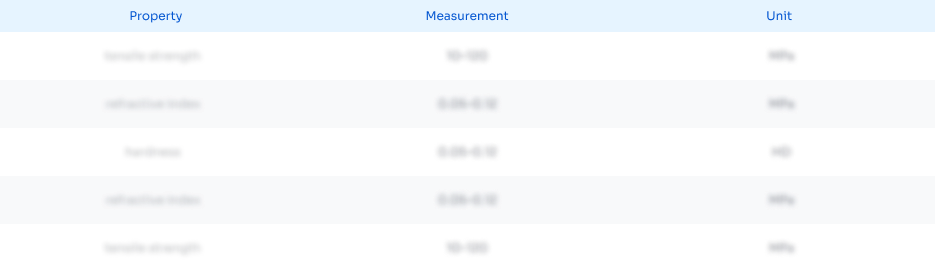
Abstract
Description
Claims
Application Information
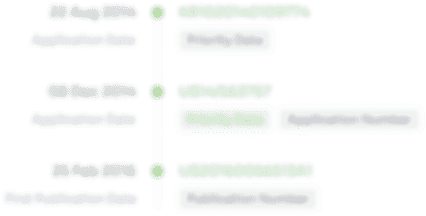
- R&D Engineer
- R&D Manager
- IP Professional
- Industry Leading Data Capabilities
- Powerful AI technology
- Patent DNA Extraction
Browse by: Latest US Patents, China's latest patents, Technical Efficacy Thesaurus, Application Domain, Technology Topic.
© 2024 PatSnap. All rights reserved.Legal|Privacy policy|Modern Slavery Act Transparency Statement|Sitemap