Convolutional neural network accelerator and acceleration method
A convolutional neural network and accelerator technology, applied in the field of convolutional neural network accelerators and acceleration, can solve problems such as limited computing resources and storage resources, achieve low power consumption, satisfy performance, and improve utilization
- Summary
- Abstract
- Description
- Claims
- Application Information
AI Technical Summary
Problems solved by technology
Method used
Image
Examples
Embodiment 1
[0030] Such as Figure 1~3As shown, a convolutional neural network accelerator includes a data input control module 1, an input buffer 2, a row buffer module 3, a matrix module 4, a convolution processing unit 5, a weight input module 6, an output buffer module 7, The bias module 8 and the pooling module 9, wherein the data input control module 1 is used to receive data from the memory or the pooling module 9, and store the received input data into the input buffer 2 in a preset order; The line buffer module 3 is used to splice the input data, output multiple lines of data, and then transmit it to the convolution processing unit 5; the matrix module 4 is used to splice the input data into an input matrix, and then transmit it to the convolution processing unit 5; the weight The input module 6 is used to cache the weights and pass the weights to the convolution processing unit 5 in a preset order; the convolution processing unit 5 is used to perform convolution operations on th...
Embodiment 2
[0042] like Figure 1~3 As shown, a convolutional neural network acceleration method includes the following steps:
[0043] Step 1, preset network acceleration parameters, receive data from the memory or pooling module 9, and store the received input data into the input buffer 2 in a preset order,
[0044] Step 2: After the weight input module 6 prepares the corresponding weight, judge whether it is a 1×1 convolution operation, if yes, the data input control module 1 outputs the data of multiple channels at one time, otherwise, the data input control module 1 presses Output the data of each channel in the preset order, and then through the line buffer module 3, the data input in a single line will be output in three lines at the same time;
[0045] Step 3: Concatenate the data into a 3×3 input matrix through the matrix module 4, then perform convolution operations on the data in the input matrix and the corresponding weights, and then perform channel-wise accumulation on the ...
PUM
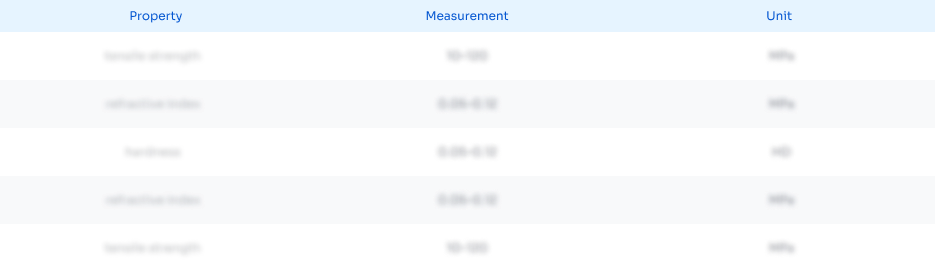
Abstract
Description
Claims
Application Information
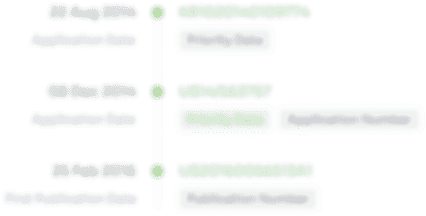
- R&D Engineer
- R&D Manager
- IP Professional
- Industry Leading Data Capabilities
- Powerful AI technology
- Patent DNA Extraction
Browse by: Latest US Patents, China's latest patents, Technical Efficacy Thesaurus, Application Domain, Technology Topic, Popular Technical Reports.
© 2024 PatSnap. All rights reserved.Legal|Privacy policy|Modern Slavery Act Transparency Statement|Sitemap|About US| Contact US: help@patsnap.com