A Human-Machine Collaborative Method for Semi-automatic Labeling of Image Object Detection Data
A target detection and human-machine collaboration technology, applied in the field of target detection, can solve the problems of reduced labeling accuracy, time-consuming, heavy workload, etc., and achieve the effect of improving running speed, excellent performance, and improving labeling fault tolerance
- Summary
- Abstract
- Description
- Claims
- Application Information
AI Technical Summary
Problems solved by technology
Method used
Image
Examples
Embodiment 1
[0032] An embodiment of the present invention provides a human-machine collaborative target detection data labeling method, the method includes the following steps:
[0033] 101: Improved target detection model Cascade R-CNN [5] (cascaded region-based convolutional neural network), remove RPN [1] (Regional candidate area network), continue to use the cascaded sub-network structure to achieve multiple corrections to the bounding box, and introduce dynamic reasoning to ensure running speed;
[0034] Furthermore, since the candidate area is directly provided by the user at this time, the RPN is no longer needed to extract the candidate area, and the RPN simplified network model is removed here, and then the cascaded network is added. This method cascades three sub-networks with the same structure for regressing bounding boxes at the back of the model. The proposals input by the latter two sub-networks are the bounding boxes output by the previous sub-network. Finally, a dynami...
Embodiment 2
[0040] The scheme in embodiment 1 is further introduced below in conjunction with specific examples and calculation formulas, see the following description for details:
[0041] 1. Data preparation
[0042] The present invention uses the general target detection dataset COCO2017 for training, which is released by Microsoft and contains more than 100,000 pictures, which can be used for multiple tasks such as target detection and semantic segmentation. Among them, the target detection task contains 80 categories of different scales and shapes.
[0043] 2. Improvement of the model
[0044] For an input picture I, this HMC R-CNN adopts 3 cascaded RoI Heads in Cascade R-CNN (the sub-network used to classify and return candidate regions in Faster R-CNN, well known to those skilled in the art ) structure, the three RoI Heads use the function g 1 , g 2 , g 3 To indicate that the backbone network (backbone) used to extract image features is recorded as a function f, and the candidat...
Embodiment 3
[0093] The experimental result 1 that the embodiment of the present invention adopts is as figure 1 and figure 2 As shown, these two pictures respectively reflect the IoU distribution between the candidate area and the final output and the ground truth bounding box, showing that the improved model of this method can effectively correct the pseudo-random candidate area, and the IoU of the candidate area after the model is processed The distribution is clearly clustered towards the part with higher IoU.
[0094] The experimental results 2 used in the embodiment of the present invention are shown in Table 1. This result shows the results of HMC R-CNN trained using the training scheme described in this method under the test conditions described in this method. Compared with the results of Cascade R-CNN on the test set of COCO2017, the performance has been very significant. improvement. Especially the detection improvement for smaller targets is particularly obvious, with more ...
PUM
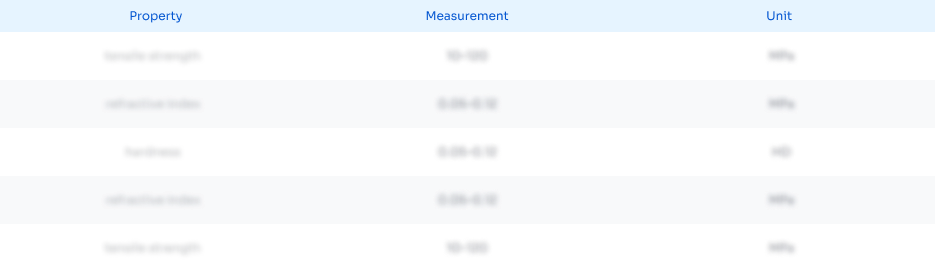
Abstract
Description
Claims
Application Information
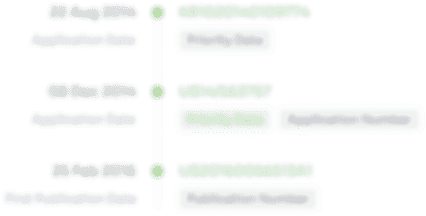
- R&D Engineer
- R&D Manager
- IP Professional
- Industry Leading Data Capabilities
- Powerful AI technology
- Patent DNA Extraction
Browse by: Latest US Patents, China's latest patents, Technical Efficacy Thesaurus, Application Domain, Technology Topic, Popular Technical Reports.
© 2024 PatSnap. All rights reserved.Legal|Privacy policy|Modern Slavery Act Transparency Statement|Sitemap|About US| Contact US: help@patsnap.com