Implementation method of end-to-end functional pulse model based on spiking neural network
A technology of a spiking neural network and an implementation method, which is applied to the implementation of an end-to-end functional spiking model, can solve problems such as insufficient coding efficiency, and achieve the effects of simple training, improved classification accuracy, training speed, and good performance.
- Summary
- Abstract
- Description
- Claims
- Application Information
AI Technical Summary
Problems solved by technology
Method used
Image
Examples
Embodiment 1
[0054] Such as figure 1 As shown, this embodiment provides a method for implementing an end-to-end functional impulse model based on the impulse neural network, including the following steps:
[0055] S1: Define input pulse binary group D i and the desired output pulse pair D d , in order to realize end-to-end learning, the present embodiment adopts a novel input information storage method, that is, utilizes the binary group D=(t, I) to represent the pulse information, where t is the pulse transmission time, and I is the pulse intensity;
[0056] Input Pulse Binary D i for
[0057] Desired output pulse binary D d for
[0058] Among them, M is the number of input pulses, N is the number of expected output pulses, I i Indicates the input pulse intensity, I d Indicates the pulse strength of the expected output at the input terminal;
[0059] S2: Construction of functional impulse response functions and dynamic synaptic functions for calculating the potential P of post...
Embodiment 2
[0079] Such as figure 2 As shown, the present embodiment is further optimized on the basis of embodiment 1, specifically:
[0080] In said S4, the functional impulse model is trained using the backpropagation algorithm, specifically:
[0081] S4.1: Initialize the training times variable step to 0;
[0082] S4.2: Select a part of training data in the source domain data set, that is, a batch;
[0083] S4.3: Obtain the predicted expected value of the output through the forward propagation algorithm;
[0084] S4.4: Calculate the training loss value, update the weight matrix W of the information transmission coefficient I in the functional spike model, the bias b of the information transmission coefficient I, and the trainable value of the current neuron pulse amplitude at each time point through the backpropagation algorithm Function parameter U and other parameters;
[0085] S4.5: Judging whether it is lower than the loss expectation value, if yes, the training ends, otherwi...
PUM
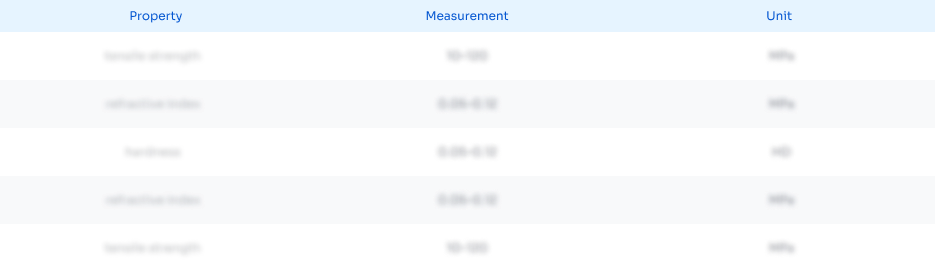
Abstract
Description
Claims
Application Information
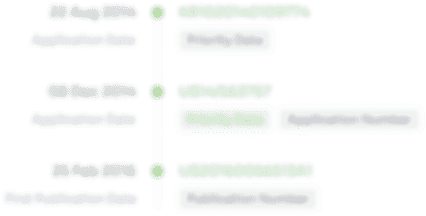
- R&D Engineer
- R&D Manager
- IP Professional
- Industry Leading Data Capabilities
- Powerful AI technology
- Patent DNA Extraction
Browse by: Latest US Patents, China's latest patents, Technical Efficacy Thesaurus, Application Domain, Technology Topic, Popular Technical Reports.
© 2024 PatSnap. All rights reserved.Legal|Privacy policy|Modern Slavery Act Transparency Statement|Sitemap|About US| Contact US: help@patsnap.com