Heterogeneous network perception model division and task placement method in pipelined distributed deep learning
A deep learning and model division technology, applied in the field of distributed computing, can solve problems such as inability to adapt to the heterogeneity of the GPU cluster network, and achieve the effect of improving the training speed
- Summary
- Abstract
- Description
- Claims
- Application Information
AI Technical Summary
Problems solved by technology
Method used
Image
Examples
Embodiment Construction
[0022] The present invention will be further described in detail below in conjunction with the accompanying drawings and specific embodiments.
[0023] The present invention is mainly carried out in the GPU cluster environment with heterogeneous network topology.
[0024] figure 1 It shows the overall architecture diagram, which mainly includes GPU server nodes connected by heterogeneous network. Heterogeneity is reflected in two aspects: heterogeneity of GPU connection methods between nodes and within nodes, and heterogeneity of connection bandwidth between nodes. Usually, the connection of GPUs is as follows: the internal nodes are connected through PCIe, and the nodes are connected through Ethernet / Infiniband. Install the CUDA library and cuDNN library on each GPU, and use the PyTorch framework to perform calculations.
[0025] figure 2 Represents the overall flow chart. First, for neural network applications, the characterization is performed layer by layer, and the cu...
PUM
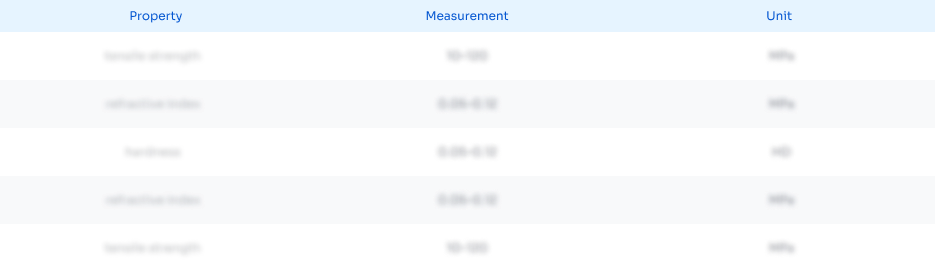
Abstract
Description
Claims
Application Information
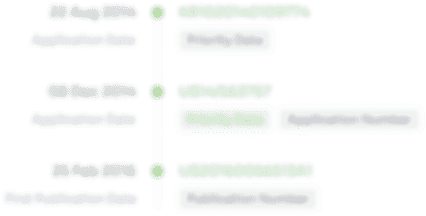
- R&D Engineer
- R&D Manager
- IP Professional
- Industry Leading Data Capabilities
- Powerful AI technology
- Patent DNA Extraction
Browse by: Latest US Patents, China's latest patents, Technical Efficacy Thesaurus, Application Domain, Technology Topic, Popular Technical Reports.
© 2024 PatSnap. All rights reserved.Legal|Privacy policy|Modern Slavery Act Transparency Statement|Sitemap|About US| Contact US: help@patsnap.com