Sequence labeling model training method, electronic medical record processing method and related device
A technology for sequence labeling and model training, which is applied in electrical digital data processing, neural learning methods, biological neural network models, etc. It can solve the problem of low sequence labeling accuracy and achieve the effect of improving accuracy.
- Summary
- Abstract
- Description
- Claims
- Application Information
AI Technical Summary
Problems solved by technology
Method used
Image
Examples
Embodiment approach
[0046]As an implementation, the feature extraction network may include a first convolutional network layer and an attention layer, the first convolutional network layer may include CNN and an improved CNN, and the improved CNN may include, but not limited to, DCNN, IDCNN, DepthwiseConvolution (depth convolution), PointwiseConvolution (pointwise convolution), Group Convolution (group convolution), etc.
[0047] As another implementation, the feature extraction network can also include a first convolutional network layer, an attention layer, and a second convolutional network layer, and both the first convolutional network layer and the second convolutional network layer can include CNN and improved CNN, the improved CNN can include, but not limited to, DCNN, IDCNN, Depthwise Convolution (depth convolution), PointwiseConvolution (pointwise convolution), Group Convolution (group convolution), etc., the following embodiments use the first convolution Both the network layer and the...
PUM
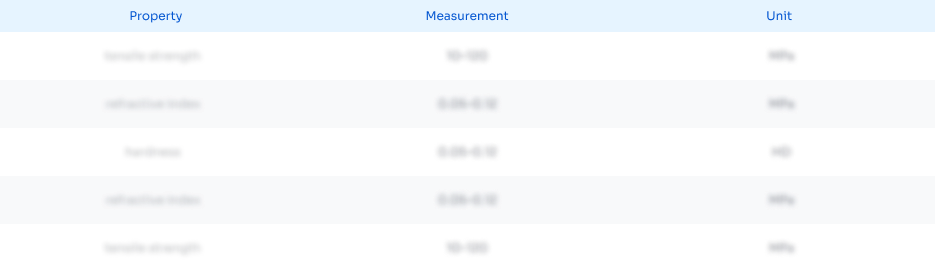
Abstract
Description
Claims
Application Information
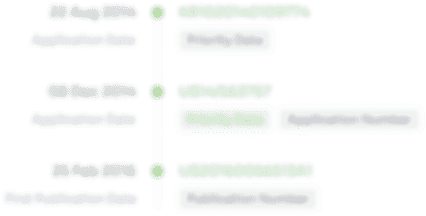
- Generate Ideas
- Intellectual Property
- Life Sciences
- Materials
- Tech Scout
- Unparalleled Data Quality
- Higher Quality Content
- 60% Fewer Hallucinations
Browse by: Latest US Patents, China's latest patents, Technical Efficacy Thesaurus, Application Domain, Technology Topic, Popular Technical Reports.
© 2025 PatSnap. All rights reserved.Legal|Privacy policy|Modern Slavery Act Transparency Statement|Sitemap|About US| Contact US: help@patsnap.com