Small data cross-domain action recognition method based on double-chain deep double-flow network
A technology of action recognition and flow network, applied in the field of computer vision and pattern recognition, can solve problems such as inability to effectively solve cross-domain tasks, inability to effectively solve cross-domain problems, inconsistent data distribution, etc., to achieve efficient action recognition performance and improve The effect of generalization ability and fast model convergence speed
- Summary
- Abstract
- Description
- Claims
- Application Information
AI Technical Summary
Problems solved by technology
Method used
Image
Examples
Embodiment 1
[0043] Such as figure 1 As shown, it is an operation flowchart of a small data cross-domain action recognition method based on a double-chain deep double-stream network of the present invention. The operation steps of the method include:
[0044] Step 10 Video Preprocessing
[0045] Due to the small number of samples in the target domain data set, the generalization ability of the model is poor, the model cannot fit the target domain data well, and the most difficult to identify sample selection and sample pair generation methods can fully solve the above problems; for example, the source domain s There are M samples {s 1 ...s i ...s M}, the target domain t has O samples {t 1 ...t i ...t O}, select the C same classes shared by both {y 1 ...y i ...y C} samples; then select the most difficult to identify samples of this class from the samples of each class in the target domain, and select the N most difficult to identify samples from all classes; through the label, the ...
PUM
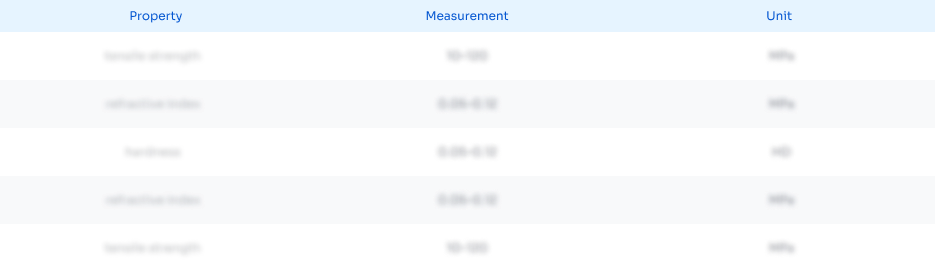
Abstract
Description
Claims
Application Information
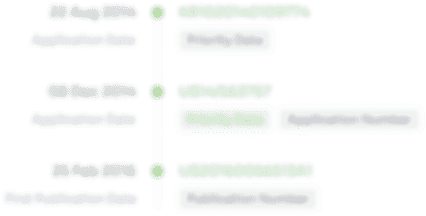
- R&D Engineer
- R&D Manager
- IP Professional
- Industry Leading Data Capabilities
- Powerful AI technology
- Patent DNA Extraction
Browse by: Latest US Patents, China's latest patents, Technical Efficacy Thesaurus, Application Domain, Technology Topic, Popular Technical Reports.
© 2024 PatSnap. All rights reserved.Legal|Privacy policy|Modern Slavery Act Transparency Statement|Sitemap|About US| Contact US: help@patsnap.com