Semi-supervised renal artery segmentation method based on dense bias network and auto-encoder
A self-encoder and bias network technology, applied in the field of image processing, can solve problems such as difficult network training, easy over-fitting, and class imbalance
- Summary
- Abstract
- Description
- Claims
- Application Information
AI Technical Summary
Problems solved by technology
Method used
Image
Examples
Embodiment Construction
[0042] Embodiments of the present invention will be described below in conjunction with the accompanying drawings.
[0043] like image 3 As shown, the present invention designs a semi-supervised renal artery segmentation method based on dense bias network and autoencoder, and uses the three-dimensional dense bias network constructed by dense bias connection technology to process abdominal CT angiography images to obtain renal artery Segmentation mask, this method specifically comprises the following steps:
[0044] Step (1), for the existing abdominal CT angiography image, segment the kidney area in the image to obtain the image of the region of interest, mark the renal artery in part of the image of the region of interest to obtain the real mask of the renal artery, and form a supervision Training data set, the remaining ROI images are formed into an unsupervised training data set, the specific process is as follows:
[0045] Step (101), manually acquiring an image of a re...
PUM
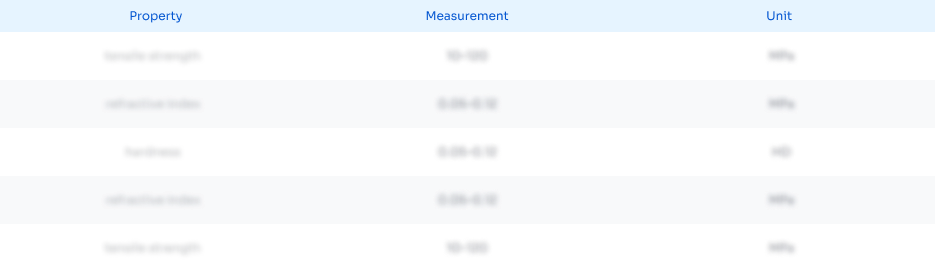
Abstract
Description
Claims
Application Information
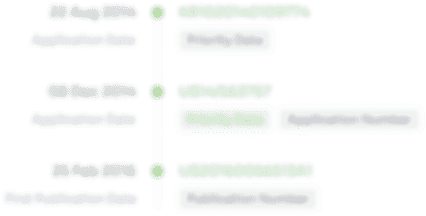
- R&D Engineer
- R&D Manager
- IP Professional
- Industry Leading Data Capabilities
- Powerful AI technology
- Patent DNA Extraction
Browse by: Latest US Patents, China's latest patents, Technical Efficacy Thesaurus, Application Domain, Technology Topic, Popular Technical Reports.
© 2024 PatSnap. All rights reserved.Legal|Privacy policy|Modern Slavery Act Transparency Statement|Sitemap|About US| Contact US: help@patsnap.com