Pathological classification method and system based on multi-modal deep learning
A technology of deep learning and classification method, which is applied in the fields of computer vision and image processing, and can solve the problem of low classification accuracy of benign and malignant breast cancer
- Summary
- Abstract
- Description
- Claims
- Application Information
AI Technical Summary
Problems solved by technology
Method used
Image
Examples
Embodiment Construction
[0029] This application proposes a data fusion method to simulate pathological diagnosis tasks. From the perspective of multimodal data fusion, try to combine pathological images in EMR with structured data to further improve the accuracy of breast cancer diagnosis. This is also in line with the actual situation when pathologists read pathological images for diagnosis. When pathologists read pathological images, they will repeatedly refer to the relevant clinical structured information in the patient's EMR as a priori until the final diagnosis is made. Among them, 29 representative attributes were extracted from clinical electronic medical records through discussion with pathologists and review of medical literature related to breast cancer. These attributes are closely related to the diagnosis of breast cancer in medical theory, and these 29 attributes are routine clinical indicators, which can be directly obtained from the existing hospital information system database.
[...
PUM
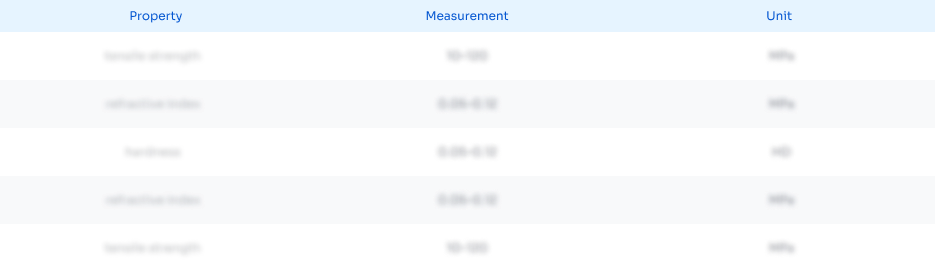
Abstract
Description
Claims
Application Information
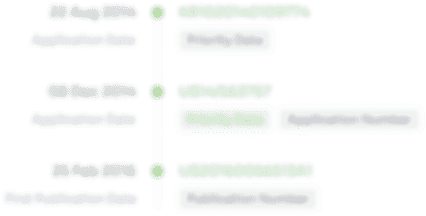
- R&D Engineer
- R&D Manager
- IP Professional
- Industry Leading Data Capabilities
- Powerful AI technology
- Patent DNA Extraction
Browse by: Latest US Patents, China's latest patents, Technical Efficacy Thesaurus, Application Domain, Technology Topic, Popular Technical Reports.
© 2024 PatSnap. All rights reserved.Legal|Privacy policy|Modern Slavery Act Transparency Statement|Sitemap|About US| Contact US: help@patsnap.com