Human motion counting method based on deep convolutional neural network
A neural network and deep convolution technology, applied in the field of human motion counting based on deep convolutional neural network, can solve problems such as inability to automatically and achieve the effect of correcting laziness
- Summary
- Abstract
- Description
- Claims
- Application Information
AI Technical Summary
Problems solved by technology
Method used
Image
Examples
Embodiment 1
[0056] In a more optimal embodiment, after the K-means clustering algorithm of step (2), also include the data enhancement process to the key action frame as training sample, the method for described data enhancement comprises translation, rotation, scale transformation and Color dithering.
[0057] The purpose of the data augmentation process is to make the features learned by the neural network robust. For this purpose, 60% of all samples collected are used as training set and 40% as testing set. In order to balance the training data, try to ensure that the number of training samples for each type of exercise is basically balanced.
Embodiment 2
[0059] In a more optimal embodiment, the used deep convolutional neural network has a structure such as image 3 As shown, compared with the traditional AlexNet neural network, two fully connected layers are reduced; only one convolutional layer B2, one pooling layer B3 and one fully connected output layer B4 are retained;
[0060] The video frame input is P1 in the figure, and the classification structure output is P5 in the figure.
[0061] Through the above simplification measures, the processing speed of the deep convolutional neural network can reach 33FPS, thereby greatly improving the calculation speed of the classification model, which can also be achieved in real time on the mobile phone.
[0062] The present invention adopts the technique of fine tune to preset the trained AlexNet weight coefficients on the ImageNet data set. The advantage is that it is easy to use the trained data without retraining the model every time, which greatly improves the practical efficie...
Embodiment 3
[0070] In a more preferred embodiment, any key action gesture sequence included in the judging process of step (5) includes at least 3 video frames.
[0071] Considering that motion occurs continuously, the output results are smoothed to reduce the error rate of recognition; and when a state appears continuously for more than 3 frames, it can be accurately judged that the key action gesture has occurred.
PUM
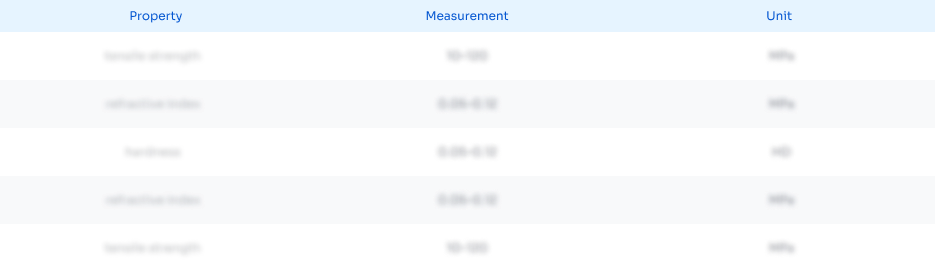
Abstract
Description
Claims
Application Information
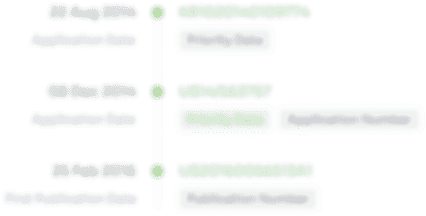
- R&D Engineer
- R&D Manager
- IP Professional
- Industry Leading Data Capabilities
- Powerful AI technology
- Patent DNA Extraction
Browse by: Latest US Patents, China's latest patents, Technical Efficacy Thesaurus, Application Domain, Technology Topic, Popular Technical Reports.
© 2024 PatSnap. All rights reserved.Legal|Privacy policy|Modern Slavery Act Transparency Statement|Sitemap|About US| Contact US: help@patsnap.com