Online self-adaptive fault monitoring and diagnosis method for process industry course
A fault monitoring and industrial process technology, applied in the direction of program control, comprehensive factory control, comprehensive factory control, etc., can solve problems such as false negatives, complex and changeable components, and insufficient response
- Summary
- Abstract
- Description
- Claims
- Application Information
AI Technical Summary
Problems solved by technology
Method used
Image
Examples
Embodiment Construction
[0049] Below in conjunction with the accompanying drawings in the implementation of the present invention, the technical solutions in the embodiments of the present invention are clearly and completely described. The described embodiments are only a part of the present invention. Based on the embodiments of the present invention, those skilled in the art All other embodiments obtained under the premise of no creative work belong to the protection scope of the present invention.
[0050] Such as Figure II Shown, the flowchart of concrete implementation of the present invention, its step comprises:
[0051] S1: Collect M (M>100) historical sample data under normal working conditions, construct a training set for the industrial process fault monitoring model, arrange the sample data in the training set by rows to form a matrix, and calculate the mean and standard deviation of the training sample set , and normalize the training set.
[0052] The data structure characteristics ...
PUM
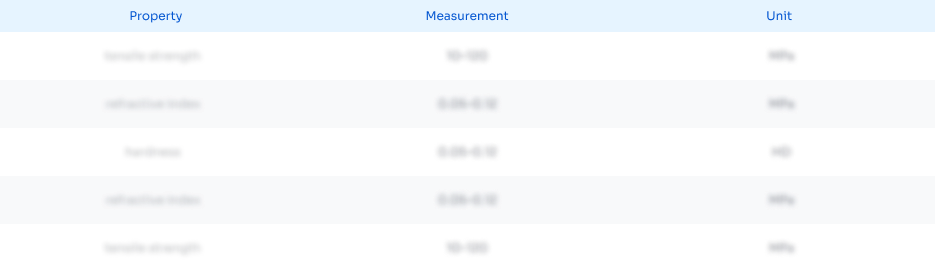
Abstract
Description
Claims
Application Information
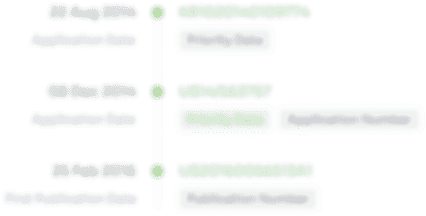
- R&D Engineer
- R&D Manager
- IP Professional
- Industry Leading Data Capabilities
- Powerful AI technology
- Patent DNA Extraction
Browse by: Latest US Patents, China's latest patents, Technical Efficacy Thesaurus, Application Domain, Technology Topic, Popular Technical Reports.
© 2024 PatSnap. All rights reserved.Legal|Privacy policy|Modern Slavery Act Transparency Statement|Sitemap|About US| Contact US: help@patsnap.com