A pedestrian re-identification method combining deep learning and metric learning
A pedestrian re-identification and metric learning technology, applied in the field of machine learning and pattern recognition, can solve the problems of unaligned pedestrian image features, unrobust changes in perspective, and insufficient discrimination, so as to achieve the effect of solving misalignment.
- Summary
- Abstract
- Description
- Claims
- Application Information
AI Technical Summary
Problems solved by technology
Method used
Image
Examples
Embodiment Construction
[0034] In order to make the purpose, technical solutions and advantages of the present invention clearer, the present invention will be described in further detail below in conjunction with the accompanying drawings.
[0035] The basic idea of the present invention is to propose a pedestrian re-identification method combining deep learning and metric learning, the flow chart of which is as follows figure 1 shown. The present invention first trains the region nomination network on the pedestrian standard pose data set, which is used to divide the entire pedestrian image into seven local deformation regions, including the head shoulder region, upper body region, lower body region, left arm region, right arm region, and left leg area, right leg area. Then combine multiple pedestrian re-identification data sets, use the local deformation area generated by the area nomination network, apply the multi-level convolution and pooling deep convolutional network, and extract the deep...
PUM
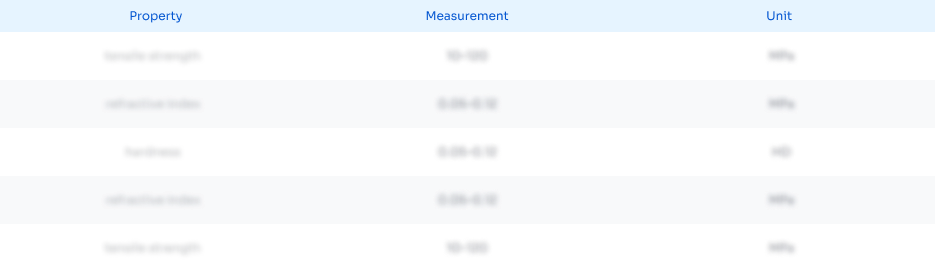
Abstract
Description
Claims
Application Information
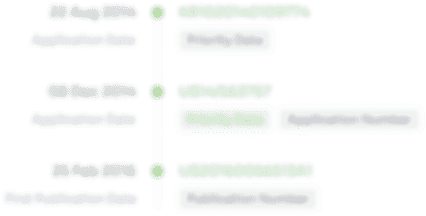
- R&D Engineer
- R&D Manager
- IP Professional
- Industry Leading Data Capabilities
- Powerful AI technology
- Patent DNA Extraction
Browse by: Latest US Patents, China's latest patents, Technical Efficacy Thesaurus, Application Domain, Technology Topic, Popular Technical Reports.
© 2024 PatSnap. All rights reserved.Legal|Privacy policy|Modern Slavery Act Transparency Statement|Sitemap|About US| Contact US: help@patsnap.com