A road scene semantic segmentation method based on convolutional neural network
A convolutional neural network and semantic segmentation technology, applied in the field of semantic segmentation of deep learning, can solve the problems of rough restored effect information, low segmentation accuracy, and reduced image feature information, so as to reduce the amount of training parameters and reduce the loss of detailed features , Improving the effect of semantic segmentation accuracy
- Summary
- Abstract
- Description
- Claims
- Application Information
AI Technical Summary
Problems solved by technology
Method used
Image
Examples
Embodiment Construction
[0051] The present invention will be further described in detail below in conjunction with the accompanying drawings and embodiments.
[0052] A method for road scene semantic segmentation based on convolutional neural network proposed by the present invention, its overall realization block diagram is as follows figure 1 As shown, it includes two processes of training phase and testing phase;
[0053] The specific steps of the described training phase process are:
[0054] Step 1_1: Select Q original road scene images and the real semantic segmentation images corresponding to each original road scene image, and form a training set, and record the qth original road scene image in the training set as {I q (i,j)}, combine the training set with {I q (i, j)} corresponding to the real semantic segmentation image is denoted as Then, the existing one-hot encoding technology (one-hot) is used to process the real semantic segmentation images corresponding to each original road scene...
PUM
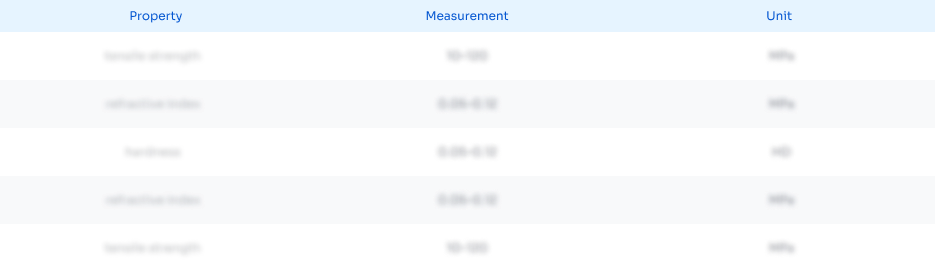
Abstract
Description
Claims
Application Information
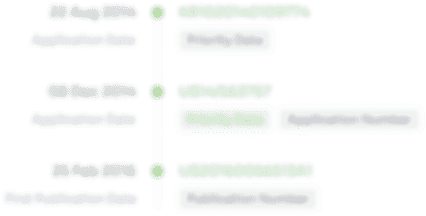
- R&D Engineer
- R&D Manager
- IP Professional
- Industry Leading Data Capabilities
- Powerful AI technology
- Patent DNA Extraction
Browse by: Latest US Patents, China's latest patents, Technical Efficacy Thesaurus, Application Domain, Technology Topic, Popular Technical Reports.
© 2024 PatSnap. All rights reserved.Legal|Privacy policy|Modern Slavery Act Transparency Statement|Sitemap|About US| Contact US: help@patsnap.com