Travel mode identification method based on big data machine learning
A travel mode and machine learning technology, applied in the field of traffic information engineering and control, can solve the problems of inaccurate description of the actual traffic volume of the road, inapplicability, etc., and achieve the effect of strong repeatability, good utilization rate, and large amount of adjustment
- Summary
- Abstract
- Description
- Claims
- Application Information
AI Technical Summary
Problems solved by technology
Method used
Image
Examples
Embodiment
[0040] Step 1, calculate and analyze districts and counties with 650,000 resident populations in the city, extract 3250 resident populations in this district as the research object according to the requirements of the present invention, then there are 3250 method acceleration detection devices in the city, and continuously collect data for 15 days As the basic data for the division of traffic modes in this district and county, the information of these 3,250 people is registered and encrypted anonymously. Combined with the mobile phone signaling data provided by the communication operator within 15 days, a total of one million sets of data have been collected. The box method is used to select data to achieve data consistency. The obtained data are as follows: figure 2 shown.
[0041] Step 2, data collection mainly includes mobile phone signaling data and acceleration detector detection data, and the mobile phone signaling data is shown in Table 1.
[0042] Table 1 Mobile phone ...
PUM
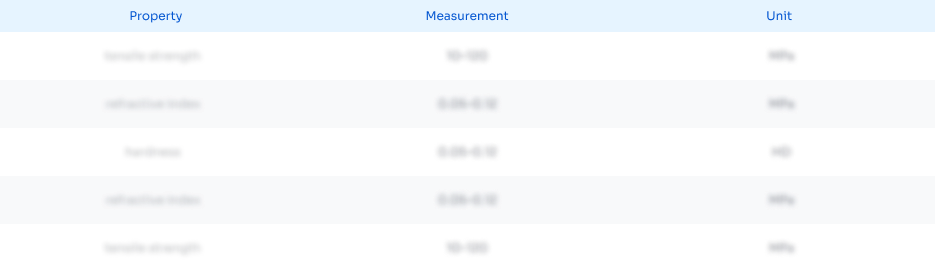
Abstract
Description
Claims
Application Information
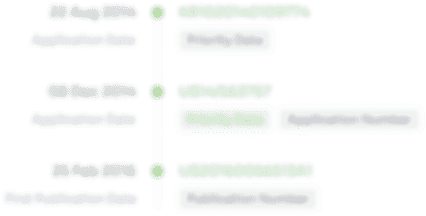
- R&D Engineer
- R&D Manager
- IP Professional
- Industry Leading Data Capabilities
- Powerful AI technology
- Patent DNA Extraction
Browse by: Latest US Patents, China's latest patents, Technical Efficacy Thesaurus, Application Domain, Technology Topic, Popular Technical Reports.
© 2024 PatSnap. All rights reserved.Legal|Privacy policy|Modern Slavery Act Transparency Statement|Sitemap|About US| Contact US: help@patsnap.com