A face attribute recognition method based on multi-instance and multi-label depth transfer learning
A technology of transfer learning and attribute recognition, which is applied in the field of face attribute recognition based on multi-instance multi-label deep transfer learning, which can solve the problems of time-consuming, low executable degree, and difficulty in determining the parameters to be adjusted, etc., to improve the speed , good effect
- Summary
- Abstract
- Description
- Claims
- Application Information
AI Technical Summary
Problems solved by technology
Method used
Image
Examples
Embodiment Construction
[0011] The present invention will be further explained below.
[0012] A face attribute recognition method based on multi-instance multi-label deep transfer learning, comprising the following steps:
[0013] Step 1: Prepare the face image data set, the face image contains the relevant face attribute labels corresponding to the face image; then extract the key point position coordinates of each face image, and use the key points for face alignment; The face image data set is randomly divided into FaceA and FaceB according to the ratio of 1:1. These two face data sets will be used in the next steps respectively.
[0014] The face image dataset selected in this example is CelebA, which has been face-aligned. There are more than 200,000 face images and 40 face attribute true values. In order to minimize the imbalance of face attribute data, this embodiment selects Arched Eyebrows, Attractive, Heavy Makeup, High Cheekbones, Male, Mouth Slightly Open, Oval Face, Pointy Nose, Smilin...
PUM
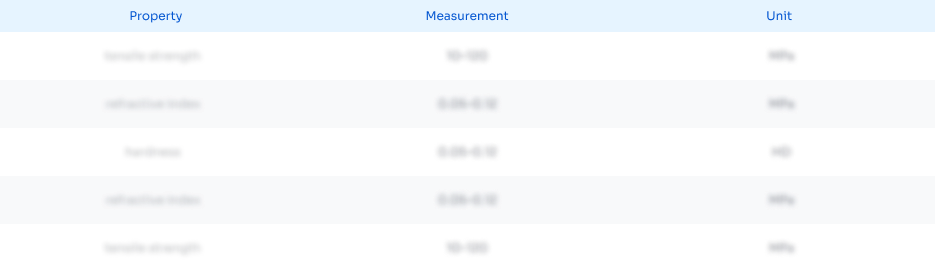
Abstract
Description
Claims
Application Information
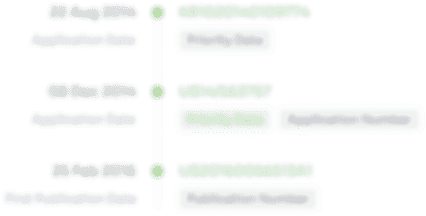
- R&D Engineer
- R&D Manager
- IP Professional
- Industry Leading Data Capabilities
- Powerful AI technology
- Patent DNA Extraction
Browse by: Latest US Patents, China's latest patents, Technical Efficacy Thesaurus, Application Domain, Technology Topic.
© 2024 PatSnap. All rights reserved.Legal|Privacy policy|Modern Slavery Act Transparency Statement|Sitemap