Deep learning algorithm based on multi-task and nearby information fusion for object detection
A technology of target detection and deep learning, applied in the direction of neural learning methods, computing, computer components, etc., can solve the problems of reducing recognition speed, fast recognition speed, and accelerating the application of convolutional neural network, so as to increase the speed and reduce convolution Calculate, shorten the effect of the process
- Summary
- Abstract
- Description
- Claims
- Application Information
AI Technical Summary
Problems solved by technology
Method used
Image
Examples
Embodiment Construction
[0021] refer to figure 1 and figure 2 , the deep learning algorithm of the multi-task based on target detection of the present invention and adjacent information fusion comprises the following steps:
[0022] Step S1: Input an initialized picture with real frames, use the pre-trained convolutional neural network to extract image features, and generate a small number of obvious target candidate frames;
[0023] Step S2: Using the image features obtained in step S1, pass the picture through the region candidate network to extract a large number of target prediction frames;
[0024] Step S3: The target prediction frame obtained in step S2 is subjected to feature extraction through the convolutional layer and feature pooling through the pooling layer, and then through the first fully connected layer to perform preliminary border regression and the direction between the target prediction frame and the real frame Prediction, preliminary target detection and classification, and ob...
PUM
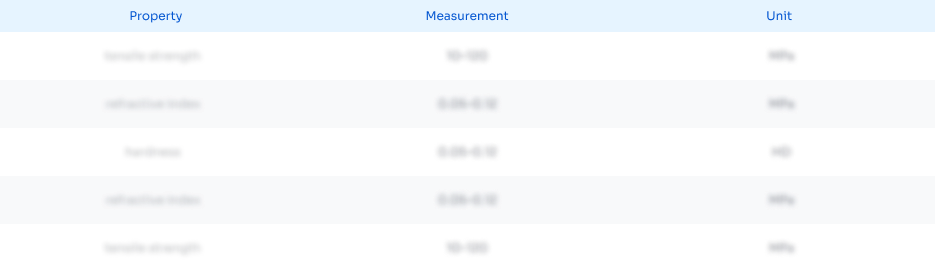
Abstract
Description
Claims
Application Information
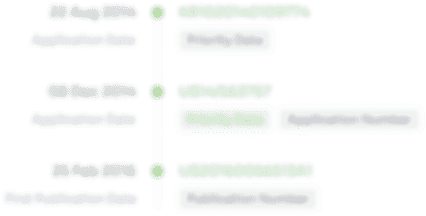
- R&D
- Intellectual Property
- Life Sciences
- Materials
- Tech Scout
- Unparalleled Data Quality
- Higher Quality Content
- 60% Fewer Hallucinations
Browse by: Latest US Patents, China's latest patents, Technical Efficacy Thesaurus, Application Domain, Technology Topic, Popular Technical Reports.
© 2025 PatSnap. All rights reserved.Legal|Privacy policy|Modern Slavery Act Transparency Statement|Sitemap|About US| Contact US: help@patsnap.com