Convolutional neural network multinuclear parallel computing method facing GPDSP
A convolutional neural network and parallel computing technology, applied in the field of deep learning, can solve problems such as accelerating convolutional neural network computing, and achieve the effects of powerful parallel computing, efficient parallel computing, and high-bandwidth vector data loading capabilities
- Summary
- Abstract
- Description
- Claims
- Application Information
AI Technical Summary
Problems solved by technology
Method used
Image
Examples
Embodiment Construction
[0041] The present invention will be further described below in conjunction with the accompanying drawings and specific preferred embodiments, but the protection scope of the present invention is not limited thereby.
[0042] The simplified memory access structure model of the GPDSP specifically adopted in this embodiment is as follows: figure 1 As shown, the system includes a CPU core unit and a DSP core unit, wherein the DSP core unit includes several 64-bit vector processing array computing units, dedicated on-chip scalar memory and vector array memory, and the on-chip shared memory shared by the CPU core unit and the DSP core unit. Storage, large-capacity off-chip DDR memory, that is, GPDSP contains multiple DSP cores of 64-bit vector processing arrays, which can simultaneously perform parallel data processing through SIMD.
[0043] Such as figure 2 As shown, the present embodiment is oriented to the convolutional neural network multi-core parallel computing method of GP...
PUM
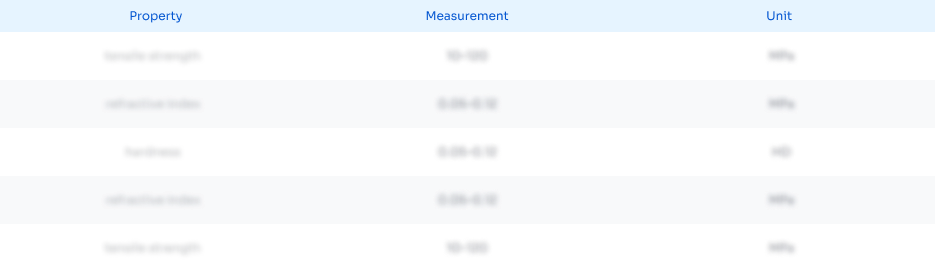
Abstract
Description
Claims
Application Information
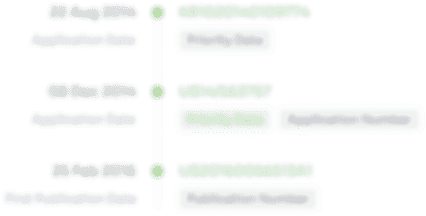
- R&D Engineer
- R&D Manager
- IP Professional
- Industry Leading Data Capabilities
- Powerful AI technology
- Patent DNA Extraction
Browse by: Latest US Patents, China's latest patents, Technical Efficacy Thesaurus, Application Domain, Technology Topic, Popular Technical Reports.
© 2024 PatSnap. All rights reserved.Legal|Privacy policy|Modern Slavery Act Transparency Statement|Sitemap|About US| Contact US: help@patsnap.com